Process CAS04 with Remote Reference¶
This notebook is a companion to the 2024 JOSS manuscript.
This notebook is shows the workflow for getting data from Earthscope for a few example stations and generating transfer functions using aurora. The data download step is based on condensed version of a tutorial in the mth5 documentation which can be found at: https://github.com/kujaku11/mth5/tree/master/docs/examples/notebooks.
[1]:
# %matplotlib notebook
# %matplotlib widget
[2]:
#Imports
import pandas as pd
import pathlib
import os
#from aurora.sandbox.mth5_channel_summary_helpers import channel_summary_to_make_mth5
#from aurora.config import BANDS_DEFAULT_FILE
from aurora.config.config_creator import ConfigCreator
from aurora.pipelines.process_mth5 import process_mth5
from mth5.mth5 import MTH5
from mth5.clients.make_mth5 import FDSN
from mth5.utils.helpers import initialize_mth5
from mtpy.processing import RunSummary, KernelDataset
/home/kkappler/software/irismt/mtpy-v2/mtpy/modeling/simpeg/recipes/inversion_2d.py:39: UserWarning: Pardiso not installed see https://github.com/simpeg/pydiso/blob/main/README.md.
warnings.warn(
[3]:
import logging, sys
logging.disable(sys.maxsize)
import warnings
warnings.filterwarnings('ignore')
Make MTH5 from IRIS Data Managment Center v0.2.0¶
This example demonstrates how to build an MTH5 from data archived at IRIS, it could work with any MT data stored at an FDSN data center (probably).
We will use the mth5.clients.FDSN
class to build the file. There is also second way using the more generic mth5.clients.MakeMTH5
class, which will be highlighted below.
Note: this example assumes that data availability (Network, Station, Channel, Start, End) are all previously known. If you do not know the data that you want to download use IRIS tools to get data availability.
Initialize a MakeMTH5 object¶
Here, we are setting the MTH5 file version to 0.2.0 so that we can have multiple surveys in a single file. Also, setting the client to “IRIS”. Here, we are using obspy.clients
tools for the request. Here are the available FDSN clients.
Note: Only the “IRIS” client has been tested.
[4]:
fdsn_object = FDSN(mth5_version='0.2.0')
fdsn_object.client = "IRIS"
Make the data inquiry as a DataFrame¶
There are a few ways to make the inquiry to request data.
Make a DataFrame by hand. Here we will make a list of entries and then create a DataFrame with the proper column names
You can create a CSV file with a row for each entry. There are some formatting that you need to be aware of. That is the column names and making sure that date-times are YYYY-MM-DDThh:mm:ss
Column Name |
Description |
---|---|
network |
|
station |
|
location |
|
channel |
|
start |
Start time (YYYY-MM-DDThh:mm:ss) UTC |
end |
End time (YYYY-MM-DDThh:mm:ss) UTC |
In the example below, the stage is set to use two stations: CAS04 and NVR08. Commented out is an example of how to add a third station, REV06
[5]:
channels = ["LFE", "LFN", "LFZ", "LQE", "LQN"]
CAS04 = ["8P", "CAS04", '2020-06-02T19:00:00', '2020-07-13T19:00:00']
NVR08 = ["8P", "NVR08", '2020-06-02T19:00:00', '2020-07-13T19:00:00']
# REV06 = ["8P", "REV06", '2020-06-02T19:00:00', '2020-07-13T19:00:00']
stations = [CAS04, NVR08,]
# stations.append(REV06)
request_list = []
for entry in stations:
for channel in channels:
request_list.append(
[entry[0], entry[1], "", channel, entry[2], entry[3]]
)
# Turn list into dataframe
request_df = pd.DataFrame(request_list, columns=fdsn_object.request_columns)
request_df
[5]:
network | station | location | channel | start | end | |
---|---|---|---|---|---|---|
0 | 8P | CAS04 | LFE | 2020-06-02T19:00:00 | 2020-07-13T19:00:00 | |
1 | 8P | CAS04 | LFN | 2020-06-02T19:00:00 | 2020-07-13T19:00:00 | |
2 | 8P | CAS04 | LFZ | 2020-06-02T19:00:00 | 2020-07-13T19:00:00 | |
3 | 8P | CAS04 | LQE | 2020-06-02T19:00:00 | 2020-07-13T19:00:00 | |
4 | 8P | CAS04 | LQN | 2020-06-02T19:00:00 | 2020-07-13T19:00:00 | |
5 | 8P | NVR08 | LFE | 2020-06-02T19:00:00 | 2020-07-13T19:00:00 | |
6 | 8P | NVR08 | LFN | 2020-06-02T19:00:00 | 2020-07-13T19:00:00 | |
7 | 8P | NVR08 | LFZ | 2020-06-02T19:00:00 | 2020-07-13T19:00:00 | |
8 | 8P | NVR08 | LQE | 2020-06-02T19:00:00 | 2020-07-13T19:00:00 | |
9 | 8P | NVR08 | LQN | 2020-06-02T19:00:00 | 2020-07-13T19:00:00 |
[6]:
%%time
mth5_filename = fdsn_object.make_mth5_from_fdsn_client(request_df, interact=False)
print(f"Created {mth5_filename}")
24:09:03T20:08:45 | INFO | line:679 |mth5.mth5 | _initialize_file | Initialized MTH5 0.2.0 file /home/kkappler/software/irismt/aurora/docs/tutorials/8P_CAS04_NVR08.h5 in mode w
24:09:03T20:09:03 | INFO | line:133 |mt_metadata.timeseries.filters.obspy_stages | create_filter_from_stage | Converting PoleZerosResponseStage electric_si_units to a CoefficientFilter.
24:09:03T20:09:03 | INFO | line:133 |mt_metadata.timeseries.filters.obspy_stages | create_filter_from_stage | Converting PoleZerosResponseStage electric_dipole_92.000 to a CoefficientFilter.
24:09:03T20:09:03 | INFO | line:133 |mt_metadata.timeseries.filters.obspy_stages | create_filter_from_stage | Converting PoleZerosResponseStage electric_si_units to a CoefficientFilter.
24:09:03T20:09:03 | INFO | line:133 |mt_metadata.timeseries.filters.obspy_stages | create_filter_from_stage | Converting PoleZerosResponseStage electric_dipole_92.000 to a CoefficientFilter.
24:09:03T20:09:03 | INFO | line:133 |mt_metadata.timeseries.filters.obspy_stages | create_filter_from_stage | Converting PoleZerosResponseStage electric_si_units to a CoefficientFilter.
24:09:03T20:09:03 | INFO | line:133 |mt_metadata.timeseries.filters.obspy_stages | create_filter_from_stage | Converting PoleZerosResponseStage electric_dipole_92.000 to a CoefficientFilter.
24:09:03T20:09:03 | INFO | line:133 |mt_metadata.timeseries.filters.obspy_stages | create_filter_from_stage | Converting PoleZerosResponseStage electric_si_units to a CoefficientFilter.
24:09:03T20:09:03 | INFO | line:133 |mt_metadata.timeseries.filters.obspy_stages | create_filter_from_stage | Converting PoleZerosResponseStage electric_dipole_92.000 to a CoefficientFilter.
24:09:03T20:09:03 | INFO | line:133 |mt_metadata.timeseries.filters.obspy_stages | create_filter_from_stage | Converting PoleZerosResponseStage electric_si_units to a CoefficientFilter.
24:09:03T20:09:03 | INFO | line:133 |mt_metadata.timeseries.filters.obspy_stages | create_filter_from_stage | Converting PoleZerosResponseStage electric_dipole_92.000 to a CoefficientFilter.
24:09:03T20:09:03 | INFO | line:133 |mt_metadata.timeseries.filters.obspy_stages | create_filter_from_stage | Converting PoleZerosResponseStage electric_si_units to a CoefficientFilter.
24:09:03T20:09:03 | INFO | line:133 |mt_metadata.timeseries.filters.obspy_stages | create_filter_from_stage | Converting PoleZerosResponseStage electric_dipole_94.000 to a CoefficientFilter.
24:09:03T20:09:03 | INFO | line:133 |mt_metadata.timeseries.filters.obspy_stages | create_filter_from_stage | Converting PoleZerosResponseStage electric_si_units to a CoefficientFilter.
24:09:03T20:09:03 | INFO | line:133 |mt_metadata.timeseries.filters.obspy_stages | create_filter_from_stage | Converting PoleZerosResponseStage electric_dipole_94.000 to a CoefficientFilter.
24:09:03T20:09:05 | INFO | line:331 |mth5.groups.base | _add_group | RunGroup a already exists, returning existing group.
24:09:03T20:09:05 | WARNING | line:645 |mth5.timeseries.run_ts | validate_metadata | start time of dataset 2020-06-02T19:00:00+00:00 does not match metadata start 2020-06-02T18:41:43+00:00 updating metatdata value to 2020-06-02T19:00:00+00:00
24:09:03T20:09:06 | WARNING | line:677 |mth5.groups.run | from_runts | Channel run.id sr1_001 != group run.id a. Setting to ch.run_metadata.id to a
24:09:03T20:09:06 | WARNING | line:677 |mth5.groups.run | from_runts | Channel run.id sr1_001 != group run.id a. Setting to ch.run_metadata.id to a
24:09:03T20:09:06 | WARNING | line:677 |mth5.groups.run | from_runts | Channel run.id sr1_001 != group run.id a. Setting to ch.run_metadata.id to a
24:09:03T20:09:06 | WARNING | line:677 |mth5.groups.run | from_runts | Channel run.id sr1_001 != group run.id a. Setting to ch.run_metadata.id to a
24:09:03T20:09:06 | WARNING | line:677 |mth5.groups.run | from_runts | Channel run.id sr1_001 != group run.id a. Setting to ch.run_metadata.id to a
24:09:03T20:09:06 | INFO | line:331 |mth5.groups.base | _add_group | RunGroup b already exists, returning existing group.
24:09:03T20:09:07 | WARNING | line:677 |mth5.groups.run | from_runts | Channel run.id sr1_001 != group run.id b. Setting to ch.run_metadata.id to b
24:09:03T20:09:07 | WARNING | line:677 |mth5.groups.run | from_runts | Channel run.id sr1_001 != group run.id b. Setting to ch.run_metadata.id to b
24:09:03T20:09:08 | WARNING | line:677 |mth5.groups.run | from_runts | Channel run.id sr1_001 != group run.id b. Setting to ch.run_metadata.id to b
24:09:03T20:09:08 | WARNING | line:677 |mth5.groups.run | from_runts | Channel run.id sr1_001 != group run.id b. Setting to ch.run_metadata.id to b
24:09:03T20:09:08 | WARNING | line:677 |mth5.groups.run | from_runts | Channel run.id sr1_001 != group run.id b. Setting to ch.run_metadata.id to b
24:09:03T20:09:08 | INFO | line:331 |mth5.groups.base | _add_group | RunGroup c already exists, returning existing group.
24:09:03T20:09:09 | WARNING | line:677 |mth5.groups.run | from_runts | Channel run.id sr1_001 != group run.id c. Setting to ch.run_metadata.id to c
24:09:03T20:09:09 | WARNING | line:677 |mth5.groups.run | from_runts | Channel run.id sr1_001 != group run.id c. Setting to ch.run_metadata.id to c
24:09:03T20:09:10 | WARNING | line:677 |mth5.groups.run | from_runts | Channel run.id sr1_001 != group run.id c. Setting to ch.run_metadata.id to c
24:09:03T20:09:10 | WARNING | line:677 |mth5.groups.run | from_runts | Channel run.id sr1_001 != group run.id c. Setting to ch.run_metadata.id to c
24:09:03T20:09:10 | WARNING | line:677 |mth5.groups.run | from_runts | Channel run.id sr1_001 != group run.id c. Setting to ch.run_metadata.id to c
24:09:03T20:09:10 | INFO | line:331 |mth5.groups.base | _add_group | RunGroup d already exists, returning existing group.
24:09:03T20:09:11 | WARNING | line:658 |mth5.timeseries.run_ts | validate_metadata | end time of dataset 2020-07-13T19:00:00+00:00 does not match metadata end 2020-07-13T21:46:12+00:00 updating metatdata value to 2020-07-13T19:00:00+00:00
24:09:03T20:09:11 | WARNING | line:677 |mth5.groups.run | from_runts | Channel run.id sr1_001 != group run.id d. Setting to ch.run_metadata.id to d
24:09:03T20:09:11 | WARNING | line:677 |mth5.groups.run | from_runts | Channel run.id sr1_001 != group run.id d. Setting to ch.run_metadata.id to d
24:09:03T20:09:11 | WARNING | line:677 |mth5.groups.run | from_runts | Channel run.id sr1_001 != group run.id d. Setting to ch.run_metadata.id to d
24:09:03T20:09:12 | WARNING | line:677 |mth5.groups.run | from_runts | Channel run.id sr1_001 != group run.id d. Setting to ch.run_metadata.id to d
24:09:03T20:09:12 | WARNING | line:677 |mth5.groups.run | from_runts | Channel run.id sr1_001 != group run.id d. Setting to ch.run_metadata.id to d
24:09:03T20:09:12 | INFO | line:331 |mth5.groups.base | _add_group | RunGroup a already exists, returning existing group.
24:09:03T20:09:12 | WARNING | line:677 |mth5.groups.run | from_runts | Channel run.id sr1_001 != group run.id a. Setting to ch.run_metadata.id to a
24:09:03T20:09:12 | WARNING | line:677 |mth5.groups.run | from_runts | Channel run.id sr1_001 != group run.id a. Setting to ch.run_metadata.id to a
24:09:03T20:09:13 | WARNING | line:677 |mth5.groups.run | from_runts | Channel run.id sr1_001 != group run.id a. Setting to ch.run_metadata.id to a
24:09:03T20:09:13 | WARNING | line:677 |mth5.groups.run | from_runts | Channel run.id sr1_001 != group run.id a. Setting to ch.run_metadata.id to a
24:09:03T20:09:13 | WARNING | line:677 |mth5.groups.run | from_runts | Channel run.id sr1_001 != group run.id a. Setting to ch.run_metadata.id to a
24:09:03T20:09:13 | INFO | line:331 |mth5.groups.base | _add_group | RunGroup b already exists, returning existing group.
24:09:03T20:09:14 | WARNING | line:677 |mth5.groups.run | from_runts | Channel run.id sr1_001 != group run.id b. Setting to ch.run_metadata.id to b
24:09:03T20:09:14 | WARNING | line:677 |mth5.groups.run | from_runts | Channel run.id sr1_001 != group run.id b. Setting to ch.run_metadata.id to b
24:09:03T20:09:14 | WARNING | line:677 |mth5.groups.run | from_runts | Channel run.id sr1_001 != group run.id b. Setting to ch.run_metadata.id to b
24:09:03T20:09:14 | WARNING | line:677 |mth5.groups.run | from_runts | Channel run.id sr1_001 != group run.id b. Setting to ch.run_metadata.id to b
24:09:03T20:09:15 | WARNING | line:677 |mth5.groups.run | from_runts | Channel run.id sr1_001 != group run.id b. Setting to ch.run_metadata.id to b
24:09:03T20:09:15 | INFO | line:331 |mth5.groups.base | _add_group | RunGroup c already exists, returning existing group.
24:09:03T20:09:15 | WARNING | line:677 |mth5.groups.run | from_runts | Channel run.id sr1_001 != group run.id c. Setting to ch.run_metadata.id to c
24:09:03T20:09:16 | WARNING | line:677 |mth5.groups.run | from_runts | Channel run.id sr1_001 != group run.id c. Setting to ch.run_metadata.id to c
24:09:03T20:09:16 | WARNING | line:677 |mth5.groups.run | from_runts | Channel run.id sr1_001 != group run.id c. Setting to ch.run_metadata.id to c
24:09:03T20:09:16 | WARNING | line:677 |mth5.groups.run | from_runts | Channel run.id sr1_001 != group run.id c. Setting to ch.run_metadata.id to c
24:09:03T20:09:16 | WARNING | line:677 |mth5.groups.run | from_runts | Channel run.id sr1_001 != group run.id c. Setting to ch.run_metadata.id to c
24:09:03T20:09:16 | INFO | line:771 |mth5.mth5 | close_mth5 | Flushing and closing /home/kkappler/software/irismt/aurora/docs/tutorials/8P_CAS04_NVR08.h5
24:09:03T20:09:16 | WARNING | line:330 |mth5.mth5 | filename | MTH5 file is not open or has not been created yet. Returning default name
Created /home/kkappler/software/irismt/aurora/docs/tutorials/8P_CAS04_NVR08.h5
CPU times: user 14.5 s, sys: 349 ms, total: 14.8 s
Wall time: 31.9 s
[7]:
mth5_path = pathlib.Path("8P_CAS04_NVR08.h5")
[8]:
m = initialize_mth5(mth5_path)
m.channel_summary.summarize()
df = m.channel_summary.to_dataframe()
df
[8]:
survey | station | run | latitude | longitude | elevation | component | start | end | n_samples | sample_rate | measurement_type | azimuth | tilt | units | has_data | hdf5_reference | run_hdf5_reference | station_hdf5_reference | |
---|---|---|---|---|---|---|---|---|---|---|---|---|---|---|---|---|---|---|---|
0 | CONUS South | CAS04 | a | 37.633351 | -121.468382 | 335.261765 | ex | 2020-06-02 19:00:00+00:00 | 2020-06-02 22:07:46+00:00 | 11267 | 1.0 | electric | 13.2 | 0.0 | digital counts | True | <HDF5 object reference> | <HDF5 object reference> | <HDF5 object reference> |
1 | CONUS South | CAS04 | a | 37.633351 | -121.468382 | 335.261765 | ey | 2020-06-02 19:00:00+00:00 | 2020-06-02 22:07:46+00:00 | 11267 | 1.0 | electric | 103.2 | 0.0 | digital counts | True | <HDF5 object reference> | <HDF5 object reference> | <HDF5 object reference> |
2 | CONUS South | CAS04 | a | 37.633351 | -121.468382 | 335.261765 | hx | 2020-06-02 19:00:00+00:00 | 2020-06-02 22:07:46+00:00 | 11267 | 1.0 | magnetic | 13.2 | 0.0 | digital counts | True | <HDF5 object reference> | <HDF5 object reference> | <HDF5 object reference> |
3 | CONUS South | CAS04 | a | 37.633351 | -121.468382 | 335.261765 | hy | 2020-06-02 19:00:00+00:00 | 2020-06-02 22:07:46+00:00 | 11267 | 1.0 | magnetic | 103.2 | 0.0 | digital counts | True | <HDF5 object reference> | <HDF5 object reference> | <HDF5 object reference> |
4 | CONUS South | CAS04 | a | 37.633351 | -121.468382 | 335.261765 | hz | 2020-06-02 19:00:00+00:00 | 2020-06-02 22:07:46+00:00 | 11267 | 1.0 | magnetic | 0.0 | 90.0 | digital counts | True | <HDF5 object reference> | <HDF5 object reference> | <HDF5 object reference> |
5 | CONUS South | CAS04 | b | 37.633351 | -121.468382 | 335.261765 | ex | 2020-06-02 22:24:55+00:00 | 2020-06-12 17:52:23+00:00 | 847649 | 1.0 | electric | 13.2 | 0.0 | digital counts | True | <HDF5 object reference> | <HDF5 object reference> | <HDF5 object reference> |
6 | CONUS South | CAS04 | b | 37.633351 | -121.468382 | 335.261765 | ey | 2020-06-02 22:24:55+00:00 | 2020-06-12 17:52:23+00:00 | 847649 | 1.0 | electric | 103.2 | 0.0 | digital counts | True | <HDF5 object reference> | <HDF5 object reference> | <HDF5 object reference> |
7 | CONUS South | CAS04 | b | 37.633351 | -121.468382 | 335.261765 | hx | 2020-06-02 22:24:55+00:00 | 2020-06-12 17:52:23+00:00 | 847649 | 1.0 | magnetic | 13.2 | 0.0 | digital counts | True | <HDF5 object reference> | <HDF5 object reference> | <HDF5 object reference> |
8 | CONUS South | CAS04 | b | 37.633351 | -121.468382 | 335.261765 | hy | 2020-06-02 22:24:55+00:00 | 2020-06-12 17:52:23+00:00 | 847649 | 1.0 | magnetic | 103.2 | 0.0 | digital counts | True | <HDF5 object reference> | <HDF5 object reference> | <HDF5 object reference> |
9 | CONUS South | CAS04 | b | 37.633351 | -121.468382 | 335.261765 | hz | 2020-06-02 22:24:55+00:00 | 2020-06-12 17:52:23+00:00 | 847649 | 1.0 | magnetic | 0.0 | 90.0 | digital counts | True | <HDF5 object reference> | <HDF5 object reference> | <HDF5 object reference> |
10 | CONUS South | CAS04 | c | 37.633351 | -121.468382 | 335.261765 | ex | 2020-06-12 18:32:17+00:00 | 2020-07-01 17:32:59+00:00 | 1638043 | 1.0 | electric | 13.2 | 0.0 | digital counts | True | <HDF5 object reference> | <HDF5 object reference> | <HDF5 object reference> |
11 | CONUS South | CAS04 | c | 37.633351 | -121.468382 | 335.261765 | ey | 2020-06-12 18:32:17+00:00 | 2020-07-01 17:32:59+00:00 | 1638043 | 1.0 | electric | 103.2 | 0.0 | digital counts | True | <HDF5 object reference> | <HDF5 object reference> | <HDF5 object reference> |
12 | CONUS South | CAS04 | c | 37.633351 | -121.468382 | 335.261765 | hx | 2020-06-12 18:32:17+00:00 | 2020-07-01 17:32:59+00:00 | 1638043 | 1.0 | magnetic | 13.2 | 0.0 | digital counts | True | <HDF5 object reference> | <HDF5 object reference> | <HDF5 object reference> |
13 | CONUS South | CAS04 | c | 37.633351 | -121.468382 | 335.261765 | hy | 2020-06-12 18:32:17+00:00 | 2020-07-01 17:32:59+00:00 | 1638043 | 1.0 | magnetic | 103.2 | 0.0 | digital counts | True | <HDF5 object reference> | <HDF5 object reference> | <HDF5 object reference> |
14 | CONUS South | CAS04 | c | 37.633351 | -121.468382 | 335.261765 | hz | 2020-06-12 18:32:17+00:00 | 2020-07-01 17:32:59+00:00 | 1638043 | 1.0 | magnetic | 0.0 | 90.0 | digital counts | True | <HDF5 object reference> | <HDF5 object reference> | <HDF5 object reference> |
15 | CONUS South | CAS04 | d | 37.633351 | -121.468382 | 335.261765 | ex | 2020-07-01 19:36:55+00:00 | 2020-07-13 19:00:00+00:00 | 1034586 | 1.0 | electric | 13.2 | 0.0 | digital counts | True | <HDF5 object reference> | <HDF5 object reference> | <HDF5 object reference> |
16 | CONUS South | CAS04 | d | 37.633351 | -121.468382 | 335.261765 | ey | 2020-07-01 19:36:55+00:00 | 2020-07-13 19:00:00+00:00 | 1034586 | 1.0 | electric | 103.2 | 0.0 | digital counts | True | <HDF5 object reference> | <HDF5 object reference> | <HDF5 object reference> |
17 | CONUS South | CAS04 | d | 37.633351 | -121.468382 | 335.261765 | hx | 2020-07-01 19:36:55+00:00 | 2020-07-13 19:00:00+00:00 | 1034586 | 1.0 | magnetic | 13.2 | 0.0 | digital counts | True | <HDF5 object reference> | <HDF5 object reference> | <HDF5 object reference> |
18 | CONUS South | CAS04 | d | 37.633351 | -121.468382 | 335.261765 | hy | 2020-07-01 19:36:55+00:00 | 2020-07-13 19:00:00+00:00 | 1034586 | 1.0 | magnetic | 103.2 | 0.0 | digital counts | True | <HDF5 object reference> | <HDF5 object reference> | <HDF5 object reference> |
19 | CONUS South | CAS04 | d | 37.633351 | -121.468382 | 335.261765 | hz | 2020-07-01 19:36:55+00:00 | 2020-07-13 19:00:00+00:00 | 1034586 | 1.0 | magnetic | 0.0 | 90.0 | digital counts | True | <HDF5 object reference> | <HDF5 object reference> | <HDF5 object reference> |
20 | CONUS South | NVR08 | a | 38.326630 | -118.082382 | 1377.902271 | ex | 2020-06-03 19:10:11+00:00 | 2020-06-03 19:57:51+00:00 | 2861 | 1.0 | electric | 12.6 | 0.0 | digital counts | True | <HDF5 object reference> | <HDF5 object reference> | <HDF5 object reference> |
21 | CONUS South | NVR08 | a | 38.326630 | -118.082382 | 1377.902271 | ey | 2020-06-03 19:10:11+00:00 | 2020-06-03 19:57:51+00:00 | 2861 | 1.0 | electric | 102.6 | 0.0 | digital counts | True | <HDF5 object reference> | <HDF5 object reference> | <HDF5 object reference> |
22 | CONUS South | NVR08 | a | 38.326630 | -118.082382 | 1377.902271 | hx | 2020-06-03 19:10:11+00:00 | 2020-06-03 19:57:51+00:00 | 2861 | 1.0 | magnetic | 12.6 | 0.0 | digital counts | True | <HDF5 object reference> | <HDF5 object reference> | <HDF5 object reference> |
23 | CONUS South | NVR08 | a | 38.326630 | -118.082382 | 1377.902271 | hy | 2020-06-03 19:10:11+00:00 | 2020-06-03 19:57:51+00:00 | 2861 | 1.0 | magnetic | 102.6 | 0.0 | digital counts | True | <HDF5 object reference> | <HDF5 object reference> | <HDF5 object reference> |
24 | CONUS South | NVR08 | a | 38.326630 | -118.082382 | 1377.902271 | hz | 2020-06-03 19:10:11+00:00 | 2020-06-03 19:57:51+00:00 | 2861 | 1.0 | magnetic | 0.0 | 90.0 | digital counts | True | <HDF5 object reference> | <HDF5 object reference> | <HDF5 object reference> |
25 | CONUS South | NVR08 | b | 38.326630 | -118.082382 | 1377.902271 | ex | 2020-06-03 20:14:13+00:00 | 2020-06-14 16:56:02+00:00 | 938510 | 1.0 | electric | 12.6 | 0.0 | digital counts | True | <HDF5 object reference> | <HDF5 object reference> | <HDF5 object reference> |
26 | CONUS South | NVR08 | b | 38.326630 | -118.082382 | 1377.902271 | ey | 2020-06-03 20:14:13+00:00 | 2020-06-14 16:56:02+00:00 | 938510 | 1.0 | electric | 102.6 | 0.0 | digital counts | True | <HDF5 object reference> | <HDF5 object reference> | <HDF5 object reference> |
27 | CONUS South | NVR08 | b | 38.326630 | -118.082382 | 1377.902271 | hx | 2020-06-03 20:14:13+00:00 | 2020-06-14 16:56:02+00:00 | 938510 | 1.0 | magnetic | 12.6 | 0.0 | digital counts | True | <HDF5 object reference> | <HDF5 object reference> | <HDF5 object reference> |
28 | CONUS South | NVR08 | b | 38.326630 | -118.082382 | 1377.902271 | hy | 2020-06-03 20:14:13+00:00 | 2020-06-14 16:56:02+00:00 | 938510 | 1.0 | magnetic | 102.6 | 0.0 | digital counts | True | <HDF5 object reference> | <HDF5 object reference> | <HDF5 object reference> |
29 | CONUS South | NVR08 | b | 38.326630 | -118.082382 | 1377.902271 | hz | 2020-06-03 20:14:13+00:00 | 2020-06-14 16:56:02+00:00 | 938510 | 1.0 | magnetic | 0.0 | 90.0 | digital counts | True | <HDF5 object reference> | <HDF5 object reference> | <HDF5 object reference> |
30 | CONUS South | NVR08 | c | 38.326630 | -118.082382 | 1377.902271 | ex | 2020-06-14 18:00:44+00:00 | 2020-06-24 15:55:46+00:00 | 856503 | 1.0 | electric | 12.6 | 0.0 | digital counts | True | <HDF5 object reference> | <HDF5 object reference> | <HDF5 object reference> |
31 | CONUS South | NVR08 | c | 38.326630 | -118.082382 | 1377.902271 | ey | 2020-06-14 18:00:44+00:00 | 2020-06-24 15:55:46+00:00 | 856503 | 1.0 | electric | 102.6 | 0.0 | digital counts | True | <HDF5 object reference> | <HDF5 object reference> | <HDF5 object reference> |
32 | CONUS South | NVR08 | c | 38.326630 | -118.082382 | 1377.902271 | hx | 2020-06-14 18:00:44+00:00 | 2020-06-24 15:55:46+00:00 | 856503 | 1.0 | magnetic | 12.6 | 0.0 | digital counts | True | <HDF5 object reference> | <HDF5 object reference> | <HDF5 object reference> |
33 | CONUS South | NVR08 | c | 38.326630 | -118.082382 | 1377.902271 | hy | 2020-06-14 18:00:44+00:00 | 2020-06-24 15:55:46+00:00 | 856503 | 1.0 | magnetic | 102.6 | 0.0 | digital counts | True | <HDF5 object reference> | <HDF5 object reference> | <HDF5 object reference> |
34 | CONUS South | NVR08 | c | 38.326630 | -118.082382 | 1377.902271 | hz | 2020-06-14 18:00:44+00:00 | 2020-06-24 15:55:46+00:00 | 856503 | 1.0 | magnetic | 0.0 | 90.0 | digital counts | True | <HDF5 object reference> | <HDF5 object reference> | <HDF5 object reference> |
Fix Survey Name¶
The survey name is extracted from the metadata provided by Earthscope
The value of the survey name was changed from “CONUS South” to “CONUS SoCal”, and the notebook was updated to reflect this, however, as of June 30, 2024, the name seems to have changed back to “CONUS South”.
To avoid problems wiht these change of nomencalature we extract the survey name as a variable
[9]:
survey_id = df["survey"].unique()[0]
survey_id
[9]:
'CONUS South'
[10]:
df.station.unique()
[10]:
array(['CAS04', 'NVR08'], dtype=object)
[11]:
mth5_run_summary = RunSummary()
mth5_run_summary.from_mth5s([mth5_path,])
run_summary = mth5_run_summary.clone()
run_summary.df
24:09:03T20:09:17 | INFO | line:771 |mth5.mth5 | close_mth5 | Flushing and closing 8P_CAS04_NVR08.h5
[11]:
channel_scale_factors | duration | end | has_data | input_channels | mth5_path | n_samples | output_channels | run | sample_rate | start | station | survey | run_hdf5_reference | station_hdf5_reference | |
---|---|---|---|---|---|---|---|---|---|---|---|---|---|---|---|
0 | {'ex': 1.0, 'ey': 1.0, 'hx': 1.0, 'hy': 1.0, '... | 11266.0 | 2020-06-02 22:07:46+00:00 | True | [hx, hy] | 8P_CAS04_NVR08.h5 | 11267 | [ex, ey, hz] | a | 1.0 | 2020-06-02 19:00:00+00:00 | CAS04 | CONUS South | <HDF5 object reference> | <HDF5 object reference> |
1 | {'ex': 1.0, 'ey': 1.0, 'hx': 1.0, 'hy': 1.0, '... | 847648.0 | 2020-06-12 17:52:23+00:00 | True | [hx, hy] | 8P_CAS04_NVR08.h5 | 847649 | [ex, ey, hz] | b | 1.0 | 2020-06-02 22:24:55+00:00 | CAS04 | CONUS South | <HDF5 object reference> | <HDF5 object reference> |
2 | {'ex': 1.0, 'ey': 1.0, 'hx': 1.0, 'hy': 1.0, '... | 1638042.0 | 2020-07-01 17:32:59+00:00 | True | [hx, hy] | 8P_CAS04_NVR08.h5 | 1638043 | [ex, ey, hz] | c | 1.0 | 2020-06-12 18:32:17+00:00 | CAS04 | CONUS South | <HDF5 object reference> | <HDF5 object reference> |
3 | {'ex': 1.0, 'ey': 1.0, 'hx': 1.0, 'hy': 1.0, '... | 1034585.0 | 2020-07-13 19:00:00+00:00 | True | [hx, hy] | 8P_CAS04_NVR08.h5 | 1034586 | [ex, ey, hz] | d | 1.0 | 2020-07-01 19:36:55+00:00 | CAS04 | CONUS South | <HDF5 object reference> | <HDF5 object reference> |
4 | {'ex': 1.0, 'ey': 1.0, 'hx': 1.0, 'hy': 1.0, '... | 2860.0 | 2020-06-03 19:57:51+00:00 | True | [hx, hy] | 8P_CAS04_NVR08.h5 | 2861 | [ex, ey, hz] | a | 1.0 | 2020-06-03 19:10:11+00:00 | NVR08 | CONUS South | <HDF5 object reference> | <HDF5 object reference> |
5 | {'ex': 1.0, 'ey': 1.0, 'hx': 1.0, 'hy': 1.0, '... | 938509.0 | 2020-06-14 16:56:02+00:00 | True | [hx, hy] | 8P_CAS04_NVR08.h5 | 938510 | [ex, ey, hz] | b | 1.0 | 2020-06-03 20:14:13+00:00 | NVR08 | CONUS South | <HDF5 object reference> | <HDF5 object reference> |
6 | {'ex': 1.0, 'ey': 1.0, 'hx': 1.0, 'hy': 1.0, '... | 856502.0 | 2020-06-24 15:55:46+00:00 | True | [hx, hy] | 8P_CAS04_NVR08.h5 | 856503 | [ex, ey, hz] | c | 1.0 | 2020-06-14 18:00:44+00:00 | NVR08 | CONUS South | <HDF5 object reference> | <HDF5 object reference> |
[12]:
coverage_short_list_columns = ["survey", 'station', 'run', 'start', 'end', ]
run_summary.df[coverage_short_list_columns]
[12]:
survey | station | run | start | end | |
---|---|---|---|---|---|
0 | CONUS South | CAS04 | a | 2020-06-02 19:00:00+00:00 | 2020-06-02 22:07:46+00:00 |
1 | CONUS South | CAS04 | b | 2020-06-02 22:24:55+00:00 | 2020-06-12 17:52:23+00:00 |
2 | CONUS South | CAS04 | c | 2020-06-12 18:32:17+00:00 | 2020-07-01 17:32:59+00:00 |
3 | CONUS South | CAS04 | d | 2020-07-01 19:36:55+00:00 | 2020-07-13 19:00:00+00:00 |
4 | CONUS South | NVR08 | a | 2020-06-03 19:10:11+00:00 | 2020-06-03 19:57:51+00:00 |
5 | CONUS South | NVR08 | b | 2020-06-03 20:14:13+00:00 | 2020-06-14 16:56:02+00:00 |
6 | CONUS South | NVR08 | c | 2020-06-14 18:00:44+00:00 | 2020-06-24 15:55:46+00:00 |
[13]:
kernel_dataset = KernelDataset()
station_id = "CAS04"
remote_reference_id = "NVR08"
kernel_dataset.from_run_summary(run_summary, station_id, remote_reference_id)
kernel_dataset.mini_summary
24:09:03T20:09:17 | INFO | line:250 |mtpy.processing.kernel_dataset | _add_columns | KernelDataset DataFrame needs column fc, adding and setting dtype to <class 'bool'>.
24:09:03T20:09:17 | INFO | line:250 |mtpy.processing.kernel_dataset | _add_columns | KernelDataset DataFrame needs column remote, adding and setting dtype to <class 'bool'>.
24:09:03T20:09:17 | INFO | line:250 |mtpy.processing.kernel_dataset | _add_columns | KernelDataset DataFrame needs column run_dataarray, adding and setting dtype to <class 'object'>.
24:09:03T20:09:17 | INFO | line:250 |mtpy.processing.kernel_dataset | _add_columns | KernelDataset DataFrame needs column stft, adding and setting dtype to <class 'object'>.
24:09:03T20:09:17 | INFO | line:250 |mtpy.processing.kernel_dataset | _add_columns | KernelDataset DataFrame needs column mth5_obj, adding and setting dtype to <class 'object'>.
[13]:
survey | station | run | start | end | duration | |
---|---|---|---|---|---|---|
0 | CONUS South | CAS04 | b | 2020-06-03 19:10:11+00:00 | 2020-06-03 19:57:51+00:00 | 2860.0 |
1 | CONUS South | NVR08 | a | 2020-06-03 19:10:11+00:00 | 2020-06-03 19:57:51+00:00 | 2860.0 |
2 | CONUS South | CAS04 | b | 2020-06-03 20:14:13+00:00 | 2020-06-12 17:52:23+00:00 | 769090.0 |
3 | CONUS South | NVR08 | b | 2020-06-03 20:14:13+00:00 | 2020-06-12 17:52:23+00:00 | 769090.0 |
4 | CONUS South | CAS04 | c | 2020-06-12 18:32:17+00:00 | 2020-06-14 16:56:02+00:00 | 167025.0 |
5 | CONUS South | NVR08 | b | 2020-06-12 18:32:17+00:00 | 2020-06-14 16:56:02+00:00 | 167025.0 |
6 | CONUS South | CAS04 | c | 2020-06-14 18:00:44+00:00 | 2020-06-24 15:55:46+00:00 | 856502.0 |
7 | CONUS South | NVR08 | c | 2020-06-14 18:00:44+00:00 | 2020-06-24 15:55:46+00:00 | 856502.0 |
[14]:
kernel_dataset = KernelDataset()
kernel_dataset.from_run_summary(run_summary, station_id, remote_reference_id)
cutoff_duration_in_seconds = 180000
kernel_dataset.drop_runs_shorter_than(cutoff_duration_in_seconds)
kernel_dataset.df[coverage_short_list_columns]
24:09:03T20:09:17 | INFO | line:250 |mtpy.processing.kernel_dataset | _add_columns | KernelDataset DataFrame needs column fc, adding and setting dtype to <class 'bool'>.
24:09:03T20:09:17 | INFO | line:250 |mtpy.processing.kernel_dataset | _add_columns | KernelDataset DataFrame needs column remote, adding and setting dtype to <class 'bool'>.
24:09:03T20:09:17 | INFO | line:250 |mtpy.processing.kernel_dataset | _add_columns | KernelDataset DataFrame needs column run_dataarray, adding and setting dtype to <class 'object'>.
24:09:03T20:09:17 | INFO | line:250 |mtpy.processing.kernel_dataset | _add_columns | KernelDataset DataFrame needs column stft, adding and setting dtype to <class 'object'>.
24:09:03T20:09:17 | INFO | line:250 |mtpy.processing.kernel_dataset | _add_columns | KernelDataset DataFrame needs column mth5_obj, adding and setting dtype to <class 'object'>.
[14]:
survey | station | run | start | end | |
---|---|---|---|---|---|
0 | CONUS South | CAS04 | b | 2020-06-03 20:14:13+00:00 | 2020-06-12 17:52:23+00:00 |
1 | CONUS South | NVR08 | b | 2020-06-03 20:14:13+00:00 | 2020-06-12 17:52:23+00:00 |
2 | CONUS South | CAS04 | c | 2020-06-14 18:00:44+00:00 | 2020-06-24 15:55:46+00:00 |
3 | CONUS South | NVR08 | c | 2020-06-14 18:00:44+00:00 | 2020-06-24 15:55:46+00:00 |
[15]:
cc = ConfigCreator()
config = cc.create_from_kernel_dataset(kernel_dataset,)
# emtf_band_file=BANDS_DEFAULT_FILE,)
24:09:03T20:09:17 | INFO | line:108 |aurora.config.config_creator | determine_band_specification_style | Bands not defined; setting to EMTF BANDS_DEFAULT_FILE
[16]:
config
[16]:
{
"processing": {
"band_setup_file": "/home/kkappler/software/irismt/aurora/aurora/config/emtf_band_setup/bs_test.cfg",
"band_specification_style": "EMTF",
"channel_nomenclature.ex": "ex",
"channel_nomenclature.ey": "ey",
"channel_nomenclature.hx": "hx",
"channel_nomenclature.hy": "hy",
"channel_nomenclature.hz": "hz",
"decimations": [
{
"decimation_level": {
"anti_alias_filter": "default",
"bands": [
{
"band": {
"center_averaging_type": "geometric",
"closed": "left",
"decimation_level": 0,
"frequency_max": 0.23828125,
"frequency_min": 0.19140625,
"index_max": 30,
"index_min": 25
}
},
{
"band": {
"center_averaging_type": "geometric",
"closed": "left",
"decimation_level": 0,
"frequency_max": 0.19140625,
"frequency_min": 0.15234375,
"index_max": 24,
"index_min": 20
}
},
{
"band": {
"center_averaging_type": "geometric",
"closed": "left",
"decimation_level": 0,
"frequency_max": 0.15234375,
"frequency_min": 0.12109375,
"index_max": 19,
"index_min": 16
}
},
{
"band": {
"center_averaging_type": "geometric",
"closed": "left",
"decimation_level": 0,
"frequency_max": 0.12109375,
"frequency_min": 0.09765625,
"index_max": 15,
"index_min": 13
}
},
{
"band": {
"center_averaging_type": "geometric",
"closed": "left",
"decimation_level": 0,
"frequency_max": 0.09765625,
"frequency_min": 0.07421875,
"index_max": 12,
"index_min": 10
}
},
{
"band": {
"center_averaging_type": "geometric",
"closed": "left",
"decimation_level": 0,
"frequency_max": 0.07421875,
"frequency_min": 0.05859375,
"index_max": 9,
"index_min": 8
}
},
{
"band": {
"center_averaging_type": "geometric",
"closed": "left",
"decimation_level": 0,
"frequency_max": 0.05859375,
"frequency_min": 0.04296875,
"index_max": 7,
"index_min": 6
}
},
{
"band": {
"center_averaging_type": "geometric",
"closed": "left",
"decimation_level": 0,
"frequency_max": 0.04296875,
"frequency_min": 0.03515625,
"index_max": 5,
"index_min": 5
}
}
],
"decimation.factor": 1.0,
"decimation.level": 0,
"decimation.method": "default",
"decimation.sample_rate": 1.0,
"estimator.engine": "RME_RR",
"estimator.estimate_per_channel": true,
"extra_pre_fft_detrend_type": "linear",
"input_channels": [
"hx",
"hy"
],
"method": "fft",
"min_num_stft_windows": 2,
"output_channels": [
"ex",
"ey",
"hz"
],
"pre_fft_detrend_type": "linear",
"prewhitening_type": "first difference",
"recoloring": true,
"reference_channels": [
"hx",
"hy"
],
"regression.max_iterations": 10,
"regression.max_redescending_iterations": 2,
"regression.minimum_cycles": 10,
"regression.r0": 1.5,
"regression.tolerance": 0.005,
"regression.u0": 2.8,
"regression.verbosity": 0,
"save_fcs": false,
"window.clock_zero_type": "ignore",
"window.num_samples": 128,
"window.overlap": 32,
"window.type": "boxcar"
}
},
{
"decimation_level": {
"anti_alias_filter": "default",
"bands": [
{
"band": {
"center_averaging_type": "geometric",
"closed": "left",
"decimation_level": 1,
"frequency_max": 0.0341796875,
"frequency_min": 0.0263671875,
"index_max": 17,
"index_min": 14
}
},
{
"band": {
"center_averaging_type": "geometric",
"closed": "left",
"decimation_level": 1,
"frequency_max": 0.0263671875,
"frequency_min": 0.0205078125,
"index_max": 13,
"index_min": 11
}
},
{
"band": {
"center_averaging_type": "geometric",
"closed": "left",
"decimation_level": 1,
"frequency_max": 0.0205078125,
"frequency_min": 0.0166015625,
"index_max": 10,
"index_min": 9
}
},
{
"band": {
"center_averaging_type": "geometric",
"closed": "left",
"decimation_level": 1,
"frequency_max": 0.0166015625,
"frequency_min": 0.0126953125,
"index_max": 8,
"index_min": 7
}
},
{
"band": {
"center_averaging_type": "geometric",
"closed": "left",
"decimation_level": 1,
"frequency_max": 0.0126953125,
"frequency_min": 0.0107421875,
"index_max": 6,
"index_min": 6
}
},
{
"band": {
"center_averaging_type": "geometric",
"closed": "left",
"decimation_level": 1,
"frequency_max": 0.0107421875,
"frequency_min": 0.0087890625,
"index_max": 5,
"index_min": 5
}
}
],
"decimation.factor": 4.0,
"decimation.level": 1,
"decimation.method": "default",
"decimation.sample_rate": 0.25,
"estimator.engine": "RME_RR",
"estimator.estimate_per_channel": true,
"extra_pre_fft_detrend_type": "linear",
"input_channels": [
"hx",
"hy"
],
"method": "fft",
"min_num_stft_windows": 2,
"output_channels": [
"ex",
"ey",
"hz"
],
"pre_fft_detrend_type": "linear",
"prewhitening_type": "first difference",
"recoloring": true,
"reference_channels": [
"hx",
"hy"
],
"regression.max_iterations": 10,
"regression.max_redescending_iterations": 2,
"regression.minimum_cycles": 10,
"regression.r0": 1.5,
"regression.tolerance": 0.005,
"regression.u0": 2.8,
"regression.verbosity": 0,
"save_fcs": false,
"window.clock_zero_type": "ignore",
"window.num_samples": 128,
"window.overlap": 32,
"window.type": "boxcar"
}
},
{
"decimation_level": {
"anti_alias_filter": "default",
"bands": [
{
"band": {
"center_averaging_type": "geometric",
"closed": "left",
"decimation_level": 2,
"frequency_max": 0.008544921875,
"frequency_min": 0.006591796875,
"index_max": 17,
"index_min": 14
}
},
{
"band": {
"center_averaging_type": "geometric",
"closed": "left",
"decimation_level": 2,
"frequency_max": 0.006591796875,
"frequency_min": 0.005126953125,
"index_max": 13,
"index_min": 11
}
},
{
"band": {
"center_averaging_type": "geometric",
"closed": "left",
"decimation_level": 2,
"frequency_max": 0.005126953125,
"frequency_min": 0.004150390625,
"index_max": 10,
"index_min": 9
}
},
{
"band": {
"center_averaging_type": "geometric",
"closed": "left",
"decimation_level": 2,
"frequency_max": 0.004150390625,
"frequency_min": 0.003173828125,
"index_max": 8,
"index_min": 7
}
},
{
"band": {
"center_averaging_type": "geometric",
"closed": "left",
"decimation_level": 2,
"frequency_max": 0.003173828125,
"frequency_min": 0.002685546875,
"index_max": 6,
"index_min": 6
}
},
{
"band": {
"center_averaging_type": "geometric",
"closed": "left",
"decimation_level": 2,
"frequency_max": 0.002685546875,
"frequency_min": 0.002197265625,
"index_max": 5,
"index_min": 5
}
}
],
"decimation.factor": 4.0,
"decimation.level": 2,
"decimation.method": "default",
"decimation.sample_rate": 0.0625,
"estimator.engine": "RME_RR",
"estimator.estimate_per_channel": true,
"extra_pre_fft_detrend_type": "linear",
"input_channels": [
"hx",
"hy"
],
"method": "fft",
"min_num_stft_windows": 2,
"output_channels": [
"ex",
"ey",
"hz"
],
"pre_fft_detrend_type": "linear",
"prewhitening_type": "first difference",
"recoloring": true,
"reference_channels": [
"hx",
"hy"
],
"regression.max_iterations": 10,
"regression.max_redescending_iterations": 2,
"regression.minimum_cycles": 10,
"regression.r0": 1.5,
"regression.tolerance": 0.005,
"regression.u0": 2.8,
"regression.verbosity": 0,
"save_fcs": false,
"window.clock_zero_type": "ignore",
"window.num_samples": 128,
"window.overlap": 32,
"window.type": "boxcar"
}
},
{
"decimation_level": {
"anti_alias_filter": "default",
"bands": [
{
"band": {
"center_averaging_type": "geometric",
"closed": "left",
"decimation_level": 3,
"frequency_max": 0.00274658203125,
"frequency_min": 0.00213623046875,
"index_max": 22,
"index_min": 18
}
},
{
"band": {
"center_averaging_type": "geometric",
"closed": "left",
"decimation_level": 3,
"frequency_max": 0.00213623046875,
"frequency_min": 0.00164794921875,
"index_max": 17,
"index_min": 14
}
},
{
"band": {
"center_averaging_type": "geometric",
"closed": "left",
"decimation_level": 3,
"frequency_max": 0.00164794921875,
"frequency_min": 0.00115966796875,
"index_max": 13,
"index_min": 10
}
},
{
"band": {
"center_averaging_type": "geometric",
"closed": "left",
"decimation_level": 3,
"frequency_max": 0.00115966796875,
"frequency_min": 0.00079345703125,
"index_max": 9,
"index_min": 7
}
},
{
"band": {
"center_averaging_type": "geometric",
"closed": "left",
"decimation_level": 3,
"frequency_max": 0.00079345703125,
"frequency_min": 0.00054931640625,
"index_max": 6,
"index_min": 5
}
}
],
"decimation.factor": 4.0,
"decimation.level": 3,
"decimation.method": "default",
"decimation.sample_rate": 0.015625,
"estimator.engine": "RME_RR",
"estimator.estimate_per_channel": true,
"extra_pre_fft_detrend_type": "linear",
"input_channels": [
"hx",
"hy"
],
"method": "fft",
"min_num_stft_windows": 2,
"output_channels": [
"ex",
"ey",
"hz"
],
"pre_fft_detrend_type": "linear",
"prewhitening_type": "first difference",
"recoloring": true,
"reference_channels": [
"hx",
"hy"
],
"regression.max_iterations": 10,
"regression.max_redescending_iterations": 2,
"regression.minimum_cycles": 10,
"regression.r0": 1.5,
"regression.tolerance": 0.005,
"regression.u0": 2.8,
"regression.verbosity": 0,
"save_fcs": false,
"window.clock_zero_type": "ignore",
"window.num_samples": 128,
"window.overlap": 32,
"window.type": "boxcar"
}
}
],
"id": "CAS04-rr_NVR08_sr1",
"stations.local.id": "CAS04",
"stations.local.mth5_path": "8P_CAS04_NVR08.h5",
"stations.local.remote": false,
"stations.local.runs": [
{
"run": {
"id": "b",
"input_channels": [
{
"channel": {
"id": "hx",
"scale_factor": 1.0
}
},
{
"channel": {
"id": "hy",
"scale_factor": 1.0
}
}
],
"output_channels": [
{
"channel": {
"id": "ex",
"scale_factor": 1.0
}
},
{
"channel": {
"id": "ey",
"scale_factor": 1.0
}
},
{
"channel": {
"id": "hz",
"scale_factor": 1.0
}
}
],
"sample_rate": 1.0,
"time_periods": [
{
"time_period": {
"end": "2020-06-12T17:52:23+00:00",
"start": "2020-06-03T20:14:13+00:00"
}
}
]
}
},
{
"run": {
"id": "c",
"input_channels": [
{
"channel": {
"id": "hx",
"scale_factor": 1.0
}
},
{
"channel": {
"id": "hy",
"scale_factor": 1.0
}
}
],
"output_channels": [
{
"channel": {
"id": "ex",
"scale_factor": 1.0
}
},
{
"channel": {
"id": "ey",
"scale_factor": 1.0
}
},
{
"channel": {
"id": "hz",
"scale_factor": 1.0
}
}
],
"sample_rate": 1.0,
"time_periods": [
{
"time_period": {
"end": "2020-06-24T15:55:46+00:00",
"start": "2020-06-14T18:00:44+00:00"
}
}
]
}
}
],
"stations.remote": [
{
"station": {
"id": "NVR08",
"mth5_path": "8P_CAS04_NVR08.h5",
"remote": true,
"runs": [
{
"run": {
"id": "b",
"input_channels": [
{
"channel": {
"id": "hx",
"scale_factor": 1.0
}
},
{
"channel": {
"id": "hy",
"scale_factor": 1.0
}
}
],
"output_channels": [
{
"channel": {
"id": "ex",
"scale_factor": 1.0
}
},
{
"channel": {
"id": "ey",
"scale_factor": 1.0
}
},
{
"channel": {
"id": "hz",
"scale_factor": 1.0
}
}
],
"sample_rate": 1.0,
"time_periods": [
{
"time_period": {
"end": "2020-06-12T17:52:23+00:00",
"start": "2020-06-03T20:14:13+00:00"
}
}
]
}
},
{
"run": {
"id": "c",
"input_channels": [
{
"channel": {
"id": "hx",
"scale_factor": 1.0
}
},
{
"channel": {
"id": "hy",
"scale_factor": 1.0
}
}
],
"output_channels": [
{
"channel": {
"id": "ex",
"scale_factor": 1.0
}
},
{
"channel": {
"id": "ey",
"scale_factor": 1.0
}
},
{
"channel": {
"id": "hz",
"scale_factor": 1.0
}
}
],
"sample_rate": 1.0,
"time_periods": [
{
"time_period": {
"end": "2020-06-24T15:55:46+00:00",
"start": "2020-06-14T18:00:44+00:00"
}
}
]
}
}
]
}
}
]
}
}
[17]:
for dec_level in config.decimations:
dec_level.window.type = "hamming"
[18]:
tf_file_base = f"{station_id}_RR{remote_reference_id}"
[19]:
show_plot = True
z_file_path = pathlib.Path(f"{tf_file_base}.zrr")
tf_cls = process_mth5(config,
kernel_dataset,
units="MT",
show_plot=show_plot,
z_file_path=z_file_path,
)
24:09:03T20:09:17 | INFO | line:277 |aurora.pipelines.transfer_function_kernel | show_processing_summary | Processing Summary Dataframe:
24:09:03T20:09:17 | INFO | line:278 |aurora.pipelines.transfer_function_kernel | show_processing_summary |
duration has_data n_samples run station survey run_hdf5_reference station_hdf5_reference fc remote stft mth5_obj dec_level dec_factor sample_rate window_duration num_samples_window num_samples num_stft_windows
0 769090.0 True 847649 b CAS04 CONUS South <HDF5 object reference> <HDF5 object reference> False False None None 0 1.0 1.000000 128.0 128 769090.0 8011.0
1 769090.0 True 847649 b CAS04 CONUS South <HDF5 object reference> <HDF5 object reference> False False None None 1 4.0 0.250000 512.0 128 192272.0 2002.0
2 769090.0 True 847649 b CAS04 CONUS South <HDF5 object reference> <HDF5 object reference> False False None None 2 4.0 0.062500 2048.0 128 48068.0 500.0
3 769090.0 True 847649 b CAS04 CONUS South <HDF5 object reference> <HDF5 object reference> False False None None 3 4.0 0.015625 8192.0 128 12017.0 124.0
4 856502.0 True 1638043 c CAS04 CONUS South <HDF5 object reference> <HDF5 object reference> False False None None 0 1.0 1.000000 128.0 128 856502.0 8921.0
5 856502.0 True 1638043 c CAS04 CONUS South <HDF5 object reference> <HDF5 object reference> False False None None 1 4.0 0.250000 512.0 128 214125.0 2230.0
6 856502.0 True 1638043 c CAS04 CONUS South <HDF5 object reference> <HDF5 object reference> False False None None 2 4.0 0.062500 2048.0 128 53531.0 557.0
7 856502.0 True 1638043 c CAS04 CONUS South <HDF5 object reference> <HDF5 object reference> False False None None 3 4.0 0.015625 8192.0 128 13382.0 139.0
8 769090.0 True 938510 b NVR08 CONUS South <HDF5 object reference> <HDF5 object reference> False True None None 0 1.0 1.000000 128.0 128 769090.0 8011.0
9 769090.0 True 938510 b NVR08 CONUS South <HDF5 object reference> <HDF5 object reference> False True None None 1 4.0 0.250000 512.0 128 192272.0 2002.0
10 769090.0 True 938510 b NVR08 CONUS South <HDF5 object reference> <HDF5 object reference> False True None None 2 4.0 0.062500 2048.0 128 48068.0 500.0
11 769090.0 True 938510 b NVR08 CONUS South <HDF5 object reference> <HDF5 object reference> False True None None 3 4.0 0.015625 8192.0 128 12017.0 124.0
12 856502.0 True 856503 c NVR08 CONUS South <HDF5 object reference> <HDF5 object reference> False True None None 0 1.0 1.000000 128.0 128 856502.0 8921.0
13 856502.0 True 856503 c NVR08 CONUS South <HDF5 object reference> <HDF5 object reference> False True None None 1 4.0 0.250000 512.0 128 214125.0 2230.0
14 856502.0 True 856503 c NVR08 CONUS South <HDF5 object reference> <HDF5 object reference> False True None None 2 4.0 0.062500 2048.0 128 53531.0 557.0
15 856502.0 True 856503 c NVR08 CONUS South <HDF5 object reference> <HDF5 object reference> False True None None 3 4.0 0.015625 8192.0 128 13382.0 139.0
24:09:03T20:09:17 | INFO | line:654 |aurora.pipelines.transfer_function_kernel | memory_check | Total memory: 62.74 GB
24:09:03T20:09:17 | INFO | line:658 |aurora.pipelines.transfer_function_kernel | memory_check | Total Bytes of Raw Data: 0.024 GB
24:09:03T20:09:17 | INFO | line:661 |aurora.pipelines.transfer_function_kernel | memory_check | Raw Data will use: 0.039 % of memory
24:09:03T20:09:17 | INFO | line:517 |aurora.pipelines.process_mth5 | process_mth5_legacy | Processing config indicates 4 decimation levels
24:09:03T20:09:17 | INFO | line:445 |aurora.pipelines.transfer_function_kernel | valid_decimations | After validation there are 4 valid decimation levels
24:09:03T20:09:18 | WARNING | line:645 |mth5.timeseries.run_ts | validate_metadata | start time of dataset 2020-06-03T20:14:13+00:00 does not match metadata start 2020-06-02T22:24:55+00:00 updating metatdata value to 2020-06-03T20:14:13+00:00
24:09:03T20:09:19 | WARNING | line:658 |mth5.timeseries.run_ts | validate_metadata | end time of dataset 2020-06-12T17:52:23+00:00 does not match metadata end 2020-06-14T16:56:02+00:00 updating metatdata value to 2020-06-12T17:52:23+00:00
24:09:03T20:09:20 | WARNING | line:645 |mth5.timeseries.run_ts | validate_metadata | start time of dataset 2020-06-14T18:00:44+00:00 does not match metadata start 2020-06-12T18:32:17+00:00 updating metatdata value to 2020-06-14T18:00:44+00:00
24:09:03T20:09:20 | WARNING | line:658 |mth5.timeseries.run_ts | validate_metadata | end time of dataset 2020-06-24T15:55:46+00:00 does not match metadata end 2020-07-01T17:32:59+00:00 updating metatdata value to 2020-06-24T15:55:46+00:00
24:09:03T20:09:22 | INFO | line:889 |mtpy.processing.kernel_dataset | initialize_dataframe_for_processing | Dataset dataframe initialized successfully
24:09:03T20:09:22 | INFO | line:143 |aurora.pipelines.transfer_function_kernel | update_dataset_df | Dataset Dataframe Updated for decimation level 0 Successfully
24:09:03T20:09:23 | INFO | line:354 |aurora.pipelines.process_mth5 | save_fourier_coefficients | Skip saving FCs. dec_level_config.save_fc = False
24:09:03T20:09:24 | INFO | line:354 |aurora.pipelines.process_mth5 | save_fourier_coefficients | Skip saving FCs. dec_level_config.save_fc = False
24:09:03T20:09:26 | INFO | line:354 |aurora.pipelines.process_mth5 | save_fourier_coefficients | Skip saving FCs. dec_level_config.save_fc = False
24:09:03T20:09:27 | INFO | line:354 |aurora.pipelines.process_mth5 | save_fourier_coefficients | Skip saving FCs. dec_level_config.save_fc = False
24:09:03T20:09:27 | INFO | line:35 |aurora.time_series.frequency_band_helpers | get_band_for_tf_estimate | Processing band 25.728968s (0.038867Hz)
24:09:03T20:09:27 | INFO | line:35 |aurora.time_series.frequency_band_helpers | get_band_for_tf_estimate | Processing band 19.929573s (0.050177Hz)
24:09:03T20:09:27 | INFO | line:35 |aurora.time_series.frequency_band_helpers | get_band_for_tf_estimate | Processing band 15.164131s (0.065945Hz)
24:09:03T20:09:28 | INFO | line:35 |aurora.time_series.frequency_band_helpers | get_band_for_tf_estimate | Processing band 11.746086s (0.085135Hz)
24:09:03T20:09:28 | INFO | line:35 |aurora.time_series.frequency_band_helpers | get_band_for_tf_estimate | Processing band 9.195791s (0.108745Hz)
24:09:03T20:09:29 | INFO | line:35 |aurora.time_series.frequency_band_helpers | get_band_for_tf_estimate | Processing band 7.362526s (0.135823Hz)
24:09:03T20:09:29 | INFO | line:35 |aurora.time_series.frequency_band_helpers | get_band_for_tf_estimate | Processing band 5.856115s (0.170762Hz)
24:09:03T20:09:29 | INFO | line:35 |aurora.time_series.frequency_band_helpers | get_band_for_tf_estimate | Processing band 4.682492s (0.213562Hz)
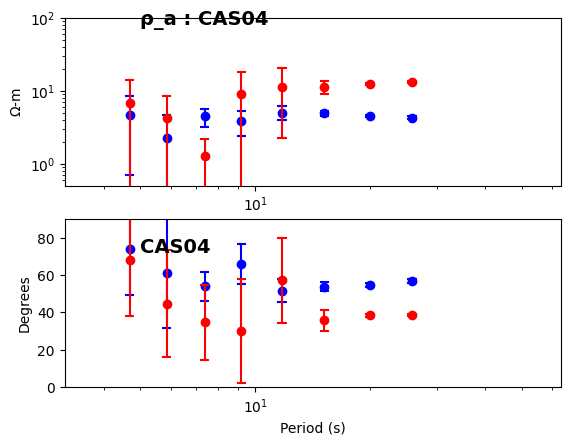
24:09:03T20:09:31 | INFO | line:124 |aurora.pipelines.transfer_function_kernel | update_dataset_df | DECIMATION LEVEL 1
24:09:03T20:09:31 | INFO | line:143 |aurora.pipelines.transfer_function_kernel | update_dataset_df | Dataset Dataframe Updated for decimation level 1 Successfully
24:09:03T20:09:32 | INFO | line:354 |aurora.pipelines.process_mth5 | save_fourier_coefficients | Skip saving FCs. dec_level_config.save_fc = False
24:09:03T20:09:32 | INFO | line:354 |aurora.pipelines.process_mth5 | save_fourier_coefficients | Skip saving FCs. dec_level_config.save_fc = False
24:09:03T20:09:33 | INFO | line:354 |aurora.pipelines.process_mth5 | save_fourier_coefficients | Skip saving FCs. dec_level_config.save_fc = False
24:09:03T20:09:33 | INFO | line:354 |aurora.pipelines.process_mth5 | save_fourier_coefficients | Skip saving FCs. dec_level_config.save_fc = False
24:09:03T20:09:33 | INFO | line:35 |aurora.time_series.frequency_band_helpers | get_band_for_tf_estimate | Processing band 102.915872s (0.009717Hz)
24:09:03T20:09:33 | INFO | line:35 |aurora.time_series.frequency_band_helpers | get_band_for_tf_estimate | Processing band 85.631182s (0.011678Hz)
24:09:03T20:09:34 | INFO | line:35 |aurora.time_series.frequency_band_helpers | get_band_for_tf_estimate | Processing band 68.881694s (0.014518Hz)
24:09:03T20:09:34 | INFO | line:35 |aurora.time_series.frequency_band_helpers | get_band_for_tf_estimate | Processing band 54.195827s (0.018452Hz)
24:09:03T20:09:34 | INFO | line:35 |aurora.time_series.frequency_band_helpers | get_band_for_tf_estimate | Processing band 43.003958s (0.023254Hz)
24:09:03T20:09:34 | INFO | line:35 |aurora.time_series.frequency_band_helpers | get_band_for_tf_estimate | Processing band 33.310722s (0.030020Hz)
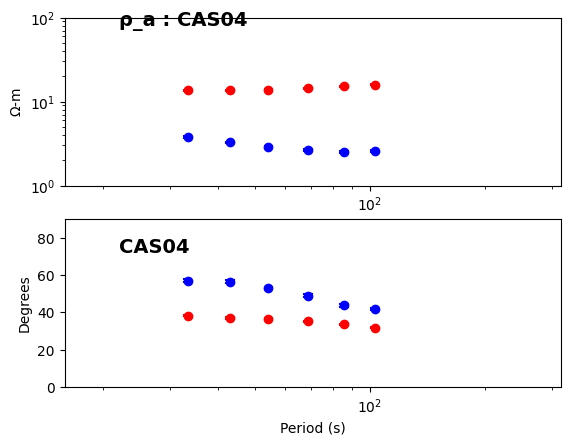
24:09:03T20:09:35 | INFO | line:124 |aurora.pipelines.transfer_function_kernel | update_dataset_df | DECIMATION LEVEL 2
24:09:03T20:09:35 | INFO | line:143 |aurora.pipelines.transfer_function_kernel | update_dataset_df | Dataset Dataframe Updated for decimation level 2 Successfully
24:09:03T20:09:35 | INFO | line:354 |aurora.pipelines.process_mth5 | save_fourier_coefficients | Skip saving FCs. dec_level_config.save_fc = False
24:09:03T20:09:36 | INFO | line:354 |aurora.pipelines.process_mth5 | save_fourier_coefficients | Skip saving FCs. dec_level_config.save_fc = False
24:09:03T20:09:36 | INFO | line:354 |aurora.pipelines.process_mth5 | save_fourier_coefficients | Skip saving FCs. dec_level_config.save_fc = False
24:09:03T20:09:37 | INFO | line:354 |aurora.pipelines.process_mth5 | save_fourier_coefficients | Skip saving FCs. dec_level_config.save_fc = False
24:09:03T20:09:37 | INFO | line:35 |aurora.time_series.frequency_band_helpers | get_band_for_tf_estimate | Processing band 411.663489s (0.002429Hz)
24:09:03T20:09:37 | INFO | line:35 |aurora.time_series.frequency_band_helpers | get_band_for_tf_estimate | Processing band 342.524727s (0.002919Hz)
24:09:03T20:09:37 | INFO | line:35 |aurora.time_series.frequency_band_helpers | get_band_for_tf_estimate | Processing band 275.526776s (0.003629Hz)
24:09:03T20:09:37 | INFO | line:35 |aurora.time_series.frequency_band_helpers | get_band_for_tf_estimate | Processing band 216.783308s (0.004613Hz)
24:09:03T20:09:37 | INFO | line:35 |aurora.time_series.frequency_band_helpers | get_band_for_tf_estimate | Processing band 172.015831s (0.005813Hz)
24:09:03T20:09:37 | INFO | line:35 |aurora.time_series.frequency_band_helpers | get_band_for_tf_estimate | Processing band 133.242890s (0.007505Hz)
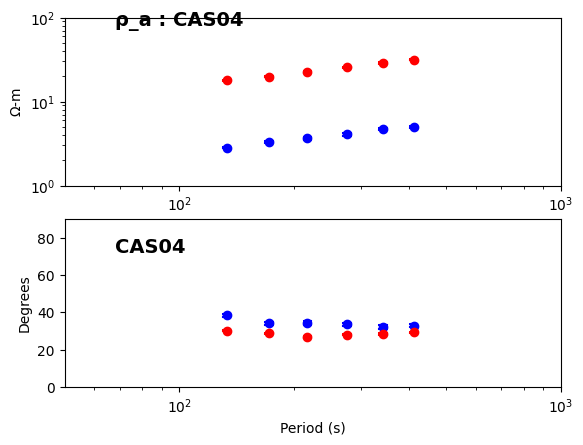
24:09:03T20:09:38 | INFO | line:124 |aurora.pipelines.transfer_function_kernel | update_dataset_df | DECIMATION LEVEL 3
24:09:03T20:09:38 | INFO | line:143 |aurora.pipelines.transfer_function_kernel | update_dataset_df | Dataset Dataframe Updated for decimation level 3 Successfully
24:09:03T20:09:38 | INFO | line:354 |aurora.pipelines.process_mth5 | save_fourier_coefficients | Skip saving FCs. dec_level_config.save_fc = False
24:09:03T20:09:38 | INFO | line:354 |aurora.pipelines.process_mth5 | save_fourier_coefficients | Skip saving FCs. dec_level_config.save_fc = False
24:09:03T20:09:39 | INFO | line:354 |aurora.pipelines.process_mth5 | save_fourier_coefficients | Skip saving FCs. dec_level_config.save_fc = False
24:09:03T20:09:39 | INFO | line:354 |aurora.pipelines.process_mth5 | save_fourier_coefficients | Skip saving FCs. dec_level_config.save_fc = False
24:09:03T20:09:39 | INFO | line:35 |aurora.time_series.frequency_band_helpers | get_band_for_tf_estimate | Processing band 1514.701336s (0.000660Hz)
24:09:03T20:09:39 | INFO | line:35 |aurora.time_series.frequency_band_helpers | get_band_for_tf_estimate | Processing band 1042.488956s (0.000959Hz)
24:09:03T20:09:39 | INFO | line:35 |aurora.time_series.frequency_band_helpers | get_band_for_tf_estimate | Processing band 723.371271s (0.001382Hz)
24:09:03T20:09:39 | INFO | line:35 |aurora.time_series.frequency_band_helpers | get_band_for_tf_estimate | Processing band 532.971560s (0.001876Hz)
24:09:03T20:09:39 | INFO | line:35 |aurora.time_series.frequency_band_helpers | get_band_for_tf_estimate | Processing band 412.837995s (0.002422Hz)
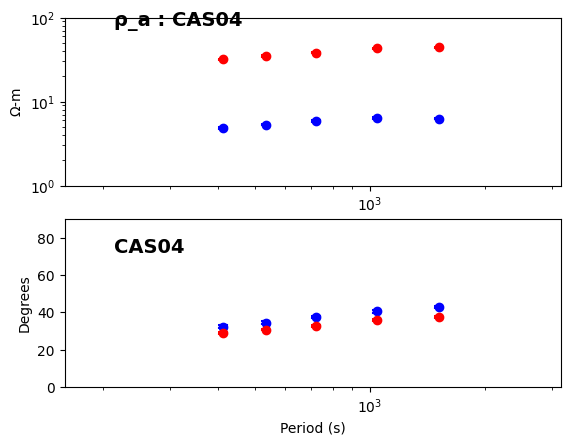
24:09:03T20:09:40 | INFO | line:771 |mth5.mth5 | close_mth5 | Flushing and closing 8P_CAS04_NVR08.h5
24:09:03T20:09:40 | INFO | line:771 |mth5.mth5 | close_mth5 | Flushing and closing 8P_CAS04_NVR08.h5
[20]:
tf_cls.write(fn=f"{tf_file_base}.xml", file_type="emtfxml")
tf_cls.write(fn=f"{tf_file_base}.edi", file_type="edi")
tf_cls.write(fn=f"{tf_file_base}.zrr", file_type="zrr")
[20]:
MT( station='CAS04', latitude=37.63, longitude=-121.47, elevation=335.26 )
[21]:
archived_z_file = pathlib.Path(f"CAS04bcd_REV06.zrr")
[22]:
from aurora.transfer_function.plot.comparison_plots import compare_two_z_files
z_file_path = "CAS04_RRNVR08.zrr"
To compare with the archived file, we need to set the coordinate system to geographic¶
The TF will be output with a header like this:
TRANSFER FUNCTIONS IN MEASUREMENT COORDINATES
********* WITH FULL ERROR COVARIANCE ********
Aurora Robust Remote Reference
station: CAS04
coordinate 37.633 -121.468 declination 13.17
number of channels 5 number of frequencies 25
orientations and tilts of each channel
1 13.20 0.00 CAS04 Hx
2 103.20 0.00 CAS04 Hy
3 0.00 90.00 CAS04 Hz
4 13.20 0.00 CAS04 Ex
5 103.20 0.00 CAS04 Ey
To remove the rotation, we can use a variety of tools, but another way is just to overwrite the orientations:
TRANSFER FUNCTIONS IN MEASUREMENT COORDINATES
********* WITH FULL ERROR COVARIANCE ********
Aurora Robust Remote Reference
station: CAS04
coordinate 37.633 -121.468 declination 13.17
number of channels 5 number of frequencies 25
orientations and tilts of each channel
1 0.00 0.00 CAS04 Hx
2 90.00 0.00 CAS04 Hy
3 0.00 90.00 CAS04 Hz
4 0.00 0.00 CAS04 Ex
5 90.00 0.00 CAS04 Ey
This is why we set angle1=13.2 degrees in the comparison plotter.
[23]:
print(z_file_path)
print(archived_z_file)
print(tf_file_base)
CAS04_RRNVR08.zrr
CAS04bcd_REV06.zrr
CAS04_RRNVR08
[24]:
compare_two_z_files(
z_file_path,
archived_z_file,
angle1=+13.2,
label1="aurora",
label2="emtf",
scale_factor1=1,
out_file=f"{tf_file_base}compare.png",
markersize=3,
rho_ylims=[1e0, 1e3],
xlims=[0.99, 2000],
rho_ax_label_size=12,
phi_ax_label_size=12
)
24:09:03T20:09:41 | INFO | line:86 |aurora.transfer_function.plot.comparison_plots | compare_two_z_files | Sacling TF scale_factor1: 1
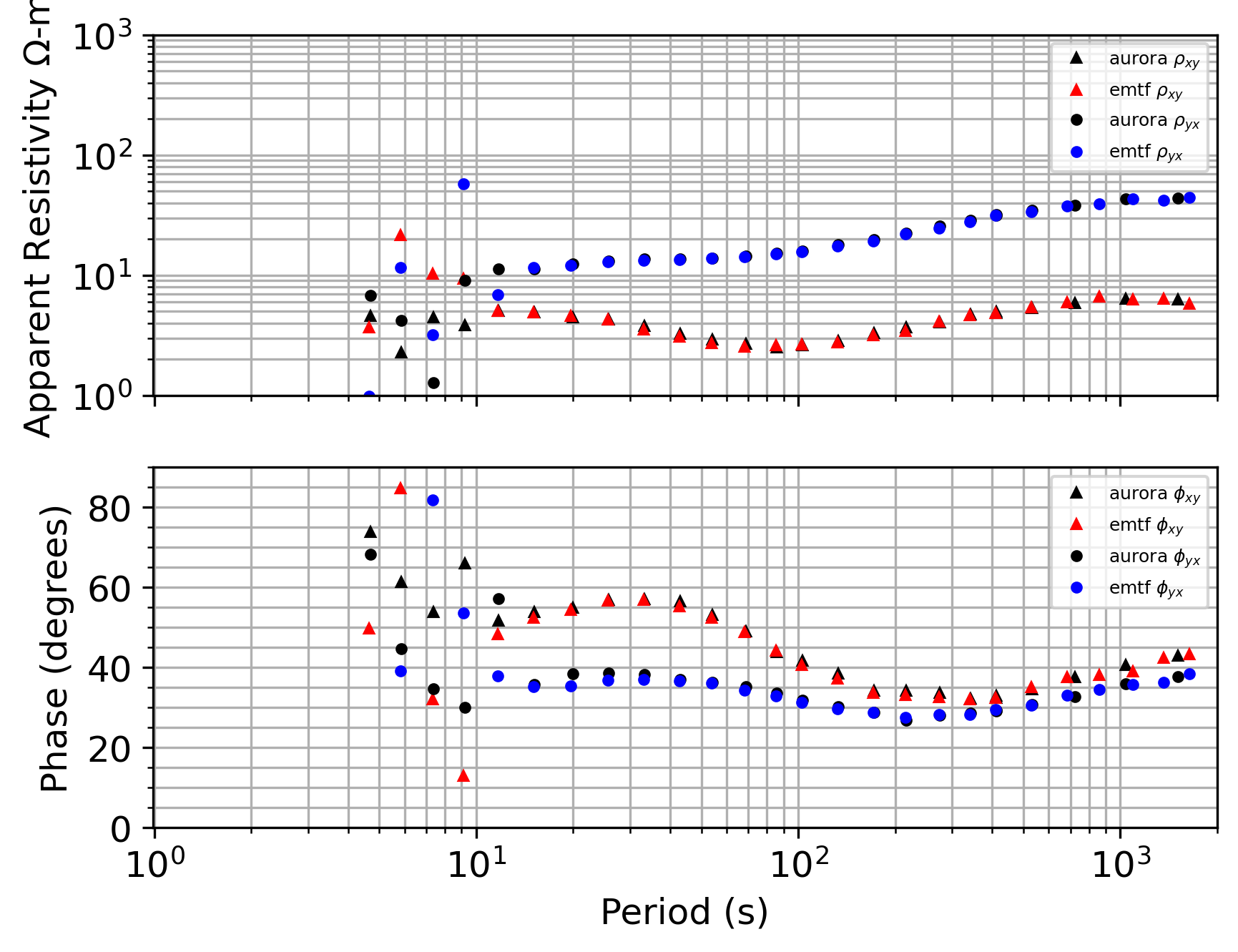
[ ]:
Part II: Logic to save FCs¶
Storage of FCs was intended to be an option to provide to users on the fly, by setting the decimation_level part of the processing config to dec_level.save_fcs = True
and dec_level.save_fcs_type = "h5"
.
This works in some cases but not in general. Details are in aurora issue #319 https://github.com/simpeg/aurora/issues/319.
The proposed solution is to generate FCs per station by processing as a single station.
We start with the Run Summary table:
[25]:
mth5_run_summary = RunSummary()
mth5_run_summary.from_mth5s([mth5_path,])
run_summary = mth5_run_summary.clone()
run_summary.df
24:09:03T20:09:42 | INFO | line:771 |mth5.mth5 | close_mth5 | Flushing and closing 8P_CAS04_NVR08.h5
[25]:
channel_scale_factors | duration | end | has_data | input_channels | mth5_path | n_samples | output_channels | run | sample_rate | start | station | survey | run_hdf5_reference | station_hdf5_reference | |
---|---|---|---|---|---|---|---|---|---|---|---|---|---|---|---|
0 | {'ex': 1.0, 'ey': 1.0, 'hx': 1.0, 'hy': 1.0, '... | 11266.0 | 2020-06-02 22:07:46+00:00 | True | [hx, hy] | 8P_CAS04_NVR08.h5 | 11267 | [ex, ey, hz] | a | 1.0 | 2020-06-02 19:00:00+00:00 | CAS04 | CONUS South | <HDF5 object reference> | <HDF5 object reference> |
1 | {'ex': 1.0, 'ey': 1.0, 'hx': 1.0, 'hy': 1.0, '... | 847648.0 | 2020-06-12 17:52:23+00:00 | True | [hx, hy] | 8P_CAS04_NVR08.h5 | 847649 | [ex, ey, hz] | b | 1.0 | 2020-06-02 22:24:55+00:00 | CAS04 | CONUS South | <HDF5 object reference> | <HDF5 object reference> |
2 | {'ex': 1.0, 'ey': 1.0, 'hx': 1.0, 'hy': 1.0, '... | 1638042.0 | 2020-07-01 17:32:59+00:00 | True | [hx, hy] | 8P_CAS04_NVR08.h5 | 1638043 | [ex, ey, hz] | c | 1.0 | 2020-06-12 18:32:17+00:00 | CAS04 | CONUS South | <HDF5 object reference> | <HDF5 object reference> |
3 | {'ex': 1.0, 'ey': 1.0, 'hx': 1.0, 'hy': 1.0, '... | 1034585.0 | 2020-07-13 19:00:00+00:00 | True | [hx, hy] | 8P_CAS04_NVR08.h5 | 1034586 | [ex, ey, hz] | d | 1.0 | 2020-07-01 19:36:55+00:00 | CAS04 | CONUS South | <HDF5 object reference> | <HDF5 object reference> |
4 | {'ex': 1.0, 'ey': 1.0, 'hx': 1.0, 'hy': 1.0, '... | 2860.0 | 2020-06-03 19:57:51+00:00 | True | [hx, hy] | 8P_CAS04_NVR08.h5 | 2861 | [ex, ey, hz] | a | 1.0 | 2020-06-03 19:10:11+00:00 | NVR08 | CONUS South | <HDF5 object reference> | <HDF5 object reference> |
5 | {'ex': 1.0, 'ey': 1.0, 'hx': 1.0, 'hy': 1.0, '... | 938509.0 | 2020-06-14 16:56:02+00:00 | True | [hx, hy] | 8P_CAS04_NVR08.h5 | 938510 | [ex, ey, hz] | b | 1.0 | 2020-06-03 20:14:13+00:00 | NVR08 | CONUS South | <HDF5 object reference> | <HDF5 object reference> |
6 | {'ex': 1.0, 'ey': 1.0, 'hx': 1.0, 'hy': 1.0, '... | 856502.0 | 2020-06-24 15:55:46+00:00 | True | [hx, hy] | 8P_CAS04_NVR08.h5 | 856503 | [ex, ey, hz] | c | 1.0 | 2020-06-14 18:00:44+00:00 | NVR08 | CONUS South | <HDF5 object reference> | <HDF5 object reference> |
[26]:
kernel_dataset = KernelDataset()
station_id = "CAS04"
remote_reference_id = None
kernel_dataset.from_run_summary(run_summary, station_id, remote_reference_id)
kernel_dataset.mini_summary
24:09:03T20:09:42 | INFO | line:250 |mtpy.processing.kernel_dataset | _add_columns | KernelDataset DataFrame needs column fc, adding and setting dtype to <class 'bool'>.
24:09:03T20:09:42 | INFO | line:250 |mtpy.processing.kernel_dataset | _add_columns | KernelDataset DataFrame needs column remote, adding and setting dtype to <class 'bool'>.
24:09:03T20:09:42 | INFO | line:250 |mtpy.processing.kernel_dataset | _add_columns | KernelDataset DataFrame needs column run_dataarray, adding and setting dtype to <class 'object'>.
24:09:03T20:09:42 | INFO | line:250 |mtpy.processing.kernel_dataset | _add_columns | KernelDataset DataFrame needs column stft, adding and setting dtype to <class 'object'>.
24:09:03T20:09:42 | INFO | line:250 |mtpy.processing.kernel_dataset | _add_columns | KernelDataset DataFrame needs column mth5_obj, adding and setting dtype to <class 'object'>.
[26]:
survey | station | run | start | end | duration | |
---|---|---|---|---|---|---|
0 | CONUS South | CAS04 | a | 2020-06-02 19:00:00+00:00 | 2020-06-02 22:07:46+00:00 | 11266.0 |
1 | CONUS South | CAS04 | b | 2020-06-02 22:24:55+00:00 | 2020-06-12 17:52:23+00:00 | 847648.0 |
2 | CONUS South | CAS04 | c | 2020-06-12 18:32:17+00:00 | 2020-07-01 17:32:59+00:00 | 1638042.0 |
3 | CONUS South | CAS04 | d | 2020-07-01 19:36:55+00:00 | 2020-07-13 19:00:00+00:00 | 1034585.0 |
Before adding the FCs, take a look at the file stats:
[27]:
file_info = os.stat(mth5_path)
print(f"file_info: \n {file_info}")
file_size_before_fc_addition = file_info.st_size
print(f"file_size_before_fc_addition {file_size_before_fc_addition}")
file_info:
os.stat_result(st_mode=33204, st_ino=89922093, st_dev=66306, st_nlink=1, st_uid=1001, st_gid=1001, st_size=107289751, st_atime=1725419382, st_mtime=1725419382, st_ctime=1725419382)
file_size_before_fc_addition 107289751
[28]:
cc = ConfigCreator()
config = cc.create_from_kernel_dataset(kernel_dataset,)
for dec_level in config.decimations:
dec_level.window.type = "hamming"
# dec_level.window.overlap = int(dec_level.window.num_samples/4)
dec_level.save_fcs = True
dec_level.save_fcs_type = "h5"
24:09:03T20:09:42 | INFO | line:108 |aurora.config.config_creator | determine_band_specification_style | Bands not defined; setting to EMTF BANDS_DEFAULT_FILE
[29]:
config
[29]:
{
"processing": {
"band_setup_file": "/home/kkappler/software/irismt/aurora/aurora/config/emtf_band_setup/bs_test.cfg",
"band_specification_style": "EMTF",
"channel_nomenclature.ex": "ex",
"channel_nomenclature.ey": "ey",
"channel_nomenclature.hx": "hx",
"channel_nomenclature.hy": "hy",
"channel_nomenclature.hz": "hz",
"decimations": [
{
"decimation_level": {
"anti_alias_filter": "default",
"bands": [
{
"band": {
"center_averaging_type": "geometric",
"closed": "left",
"decimation_level": 0,
"frequency_max": 0.23828125,
"frequency_min": 0.19140625,
"index_max": 30,
"index_min": 25
}
},
{
"band": {
"center_averaging_type": "geometric",
"closed": "left",
"decimation_level": 0,
"frequency_max": 0.19140625,
"frequency_min": 0.15234375,
"index_max": 24,
"index_min": 20
}
},
{
"band": {
"center_averaging_type": "geometric",
"closed": "left",
"decimation_level": 0,
"frequency_max": 0.15234375,
"frequency_min": 0.12109375,
"index_max": 19,
"index_min": 16
}
},
{
"band": {
"center_averaging_type": "geometric",
"closed": "left",
"decimation_level": 0,
"frequency_max": 0.12109375,
"frequency_min": 0.09765625,
"index_max": 15,
"index_min": 13
}
},
{
"band": {
"center_averaging_type": "geometric",
"closed": "left",
"decimation_level": 0,
"frequency_max": 0.09765625,
"frequency_min": 0.07421875,
"index_max": 12,
"index_min": 10
}
},
{
"band": {
"center_averaging_type": "geometric",
"closed": "left",
"decimation_level": 0,
"frequency_max": 0.07421875,
"frequency_min": 0.05859375,
"index_max": 9,
"index_min": 8
}
},
{
"band": {
"center_averaging_type": "geometric",
"closed": "left",
"decimation_level": 0,
"frequency_max": 0.05859375,
"frequency_min": 0.04296875,
"index_max": 7,
"index_min": 6
}
},
{
"band": {
"center_averaging_type": "geometric",
"closed": "left",
"decimation_level": 0,
"frequency_max": 0.04296875,
"frequency_min": 0.03515625,
"index_max": 5,
"index_min": 5
}
}
],
"decimation.factor": 1.0,
"decimation.level": 0,
"decimation.method": "default",
"decimation.sample_rate": 1.0,
"estimator.engine": "RME_RR",
"estimator.estimate_per_channel": true,
"extra_pre_fft_detrend_type": "linear",
"input_channels": [
"hx",
"hy"
],
"method": "fft",
"min_num_stft_windows": 2,
"output_channels": [
"ex",
"ey",
"hz"
],
"pre_fft_detrend_type": "linear",
"prewhitening_type": "first difference",
"recoloring": true,
"reference_channels": [
"hx",
"hy"
],
"regression.max_iterations": 10,
"regression.max_redescending_iterations": 2,
"regression.minimum_cycles": 10,
"regression.r0": 1.5,
"regression.tolerance": 0.005,
"regression.u0": 2.8,
"regression.verbosity": 0,
"save_fcs": true,
"save_fcs_type": "h5",
"window.clock_zero_type": "ignore",
"window.num_samples": 128,
"window.overlap": 32,
"window.type": "hamming"
}
},
{
"decimation_level": {
"anti_alias_filter": "default",
"bands": [
{
"band": {
"center_averaging_type": "geometric",
"closed": "left",
"decimation_level": 1,
"frequency_max": 0.0341796875,
"frequency_min": 0.0263671875,
"index_max": 17,
"index_min": 14
}
},
{
"band": {
"center_averaging_type": "geometric",
"closed": "left",
"decimation_level": 1,
"frequency_max": 0.0263671875,
"frequency_min": 0.0205078125,
"index_max": 13,
"index_min": 11
}
},
{
"band": {
"center_averaging_type": "geometric",
"closed": "left",
"decimation_level": 1,
"frequency_max": 0.0205078125,
"frequency_min": 0.0166015625,
"index_max": 10,
"index_min": 9
}
},
{
"band": {
"center_averaging_type": "geometric",
"closed": "left",
"decimation_level": 1,
"frequency_max": 0.0166015625,
"frequency_min": 0.0126953125,
"index_max": 8,
"index_min": 7
}
},
{
"band": {
"center_averaging_type": "geometric",
"closed": "left",
"decimation_level": 1,
"frequency_max": 0.0126953125,
"frequency_min": 0.0107421875,
"index_max": 6,
"index_min": 6
}
},
{
"band": {
"center_averaging_type": "geometric",
"closed": "left",
"decimation_level": 1,
"frequency_max": 0.0107421875,
"frequency_min": 0.0087890625,
"index_max": 5,
"index_min": 5
}
}
],
"decimation.factor": 4.0,
"decimation.level": 1,
"decimation.method": "default",
"decimation.sample_rate": 0.25,
"estimator.engine": "RME_RR",
"estimator.estimate_per_channel": true,
"extra_pre_fft_detrend_type": "linear",
"input_channels": [
"hx",
"hy"
],
"method": "fft",
"min_num_stft_windows": 2,
"output_channels": [
"ex",
"ey",
"hz"
],
"pre_fft_detrend_type": "linear",
"prewhitening_type": "first difference",
"recoloring": true,
"reference_channels": [
"hx",
"hy"
],
"regression.max_iterations": 10,
"regression.max_redescending_iterations": 2,
"regression.minimum_cycles": 10,
"regression.r0": 1.5,
"regression.tolerance": 0.005,
"regression.u0": 2.8,
"regression.verbosity": 0,
"save_fcs": true,
"save_fcs_type": "h5",
"window.clock_zero_type": "ignore",
"window.num_samples": 128,
"window.overlap": 32,
"window.type": "hamming"
}
},
{
"decimation_level": {
"anti_alias_filter": "default",
"bands": [
{
"band": {
"center_averaging_type": "geometric",
"closed": "left",
"decimation_level": 2,
"frequency_max": 0.008544921875,
"frequency_min": 0.006591796875,
"index_max": 17,
"index_min": 14
}
},
{
"band": {
"center_averaging_type": "geometric",
"closed": "left",
"decimation_level": 2,
"frequency_max": 0.006591796875,
"frequency_min": 0.005126953125,
"index_max": 13,
"index_min": 11
}
},
{
"band": {
"center_averaging_type": "geometric",
"closed": "left",
"decimation_level": 2,
"frequency_max": 0.005126953125,
"frequency_min": 0.004150390625,
"index_max": 10,
"index_min": 9
}
},
{
"band": {
"center_averaging_type": "geometric",
"closed": "left",
"decimation_level": 2,
"frequency_max": 0.004150390625,
"frequency_min": 0.003173828125,
"index_max": 8,
"index_min": 7
}
},
{
"band": {
"center_averaging_type": "geometric",
"closed": "left",
"decimation_level": 2,
"frequency_max": 0.003173828125,
"frequency_min": 0.002685546875,
"index_max": 6,
"index_min": 6
}
},
{
"band": {
"center_averaging_type": "geometric",
"closed": "left",
"decimation_level": 2,
"frequency_max": 0.002685546875,
"frequency_min": 0.002197265625,
"index_max": 5,
"index_min": 5
}
}
],
"decimation.factor": 4.0,
"decimation.level": 2,
"decimation.method": "default",
"decimation.sample_rate": 0.0625,
"estimator.engine": "RME_RR",
"estimator.estimate_per_channel": true,
"extra_pre_fft_detrend_type": "linear",
"input_channels": [
"hx",
"hy"
],
"method": "fft",
"min_num_stft_windows": 2,
"output_channels": [
"ex",
"ey",
"hz"
],
"pre_fft_detrend_type": "linear",
"prewhitening_type": "first difference",
"recoloring": true,
"reference_channels": [
"hx",
"hy"
],
"regression.max_iterations": 10,
"regression.max_redescending_iterations": 2,
"regression.minimum_cycles": 10,
"regression.r0": 1.5,
"regression.tolerance": 0.005,
"regression.u0": 2.8,
"regression.verbosity": 0,
"save_fcs": true,
"save_fcs_type": "h5",
"window.clock_zero_type": "ignore",
"window.num_samples": 128,
"window.overlap": 32,
"window.type": "hamming"
}
},
{
"decimation_level": {
"anti_alias_filter": "default",
"bands": [
{
"band": {
"center_averaging_type": "geometric",
"closed": "left",
"decimation_level": 3,
"frequency_max": 0.00274658203125,
"frequency_min": 0.00213623046875,
"index_max": 22,
"index_min": 18
}
},
{
"band": {
"center_averaging_type": "geometric",
"closed": "left",
"decimation_level": 3,
"frequency_max": 0.00213623046875,
"frequency_min": 0.00164794921875,
"index_max": 17,
"index_min": 14
}
},
{
"band": {
"center_averaging_type": "geometric",
"closed": "left",
"decimation_level": 3,
"frequency_max": 0.00164794921875,
"frequency_min": 0.00115966796875,
"index_max": 13,
"index_min": 10
}
},
{
"band": {
"center_averaging_type": "geometric",
"closed": "left",
"decimation_level": 3,
"frequency_max": 0.00115966796875,
"frequency_min": 0.00079345703125,
"index_max": 9,
"index_min": 7
}
},
{
"band": {
"center_averaging_type": "geometric",
"closed": "left",
"decimation_level": 3,
"frequency_max": 0.00079345703125,
"frequency_min": 0.00054931640625,
"index_max": 6,
"index_min": 5
}
}
],
"decimation.factor": 4.0,
"decimation.level": 3,
"decimation.method": "default",
"decimation.sample_rate": 0.015625,
"estimator.engine": "RME_RR",
"estimator.estimate_per_channel": true,
"extra_pre_fft_detrend_type": "linear",
"input_channels": [
"hx",
"hy"
],
"method": "fft",
"min_num_stft_windows": 2,
"output_channels": [
"ex",
"ey",
"hz"
],
"pre_fft_detrend_type": "linear",
"prewhitening_type": "first difference",
"recoloring": true,
"reference_channels": [
"hx",
"hy"
],
"regression.max_iterations": 10,
"regression.max_redescending_iterations": 2,
"regression.minimum_cycles": 10,
"regression.r0": 1.5,
"regression.tolerance": 0.005,
"regression.u0": 2.8,
"regression.verbosity": 0,
"save_fcs": true,
"save_fcs_type": "h5",
"window.clock_zero_type": "ignore",
"window.num_samples": 128,
"window.overlap": 32,
"window.type": "hamming"
}
}
],
"id": "CAS04_sr1",
"stations.local.id": "CAS04",
"stations.local.mth5_path": "8P_CAS04_NVR08.h5",
"stations.local.remote": false,
"stations.local.runs": [
{
"run": {
"id": "a",
"input_channels": [
{
"channel": {
"id": "hx",
"scale_factor": 1.0
}
},
{
"channel": {
"id": "hy",
"scale_factor": 1.0
}
}
],
"output_channels": [
{
"channel": {
"id": "ex",
"scale_factor": 1.0
}
},
{
"channel": {
"id": "ey",
"scale_factor": 1.0
}
},
{
"channel": {
"id": "hz",
"scale_factor": 1.0
}
}
],
"sample_rate": 1.0,
"time_periods": [
{
"time_period": {
"end": "2020-06-02T22:07:46+00:00",
"start": "2020-06-02T19:00:00+00:00"
}
}
]
}
},
{
"run": {
"id": "b",
"input_channels": [
{
"channel": {
"id": "hx",
"scale_factor": 1.0
}
},
{
"channel": {
"id": "hy",
"scale_factor": 1.0
}
}
],
"output_channels": [
{
"channel": {
"id": "ex",
"scale_factor": 1.0
}
},
{
"channel": {
"id": "ey",
"scale_factor": 1.0
}
},
{
"channel": {
"id": "hz",
"scale_factor": 1.0
}
}
],
"sample_rate": 1.0,
"time_periods": [
{
"time_period": {
"end": "2020-06-12T17:52:23+00:00",
"start": "2020-06-02T22:24:55+00:00"
}
}
]
}
},
{
"run": {
"id": "c",
"input_channels": [
{
"channel": {
"id": "hx",
"scale_factor": 1.0
}
},
{
"channel": {
"id": "hy",
"scale_factor": 1.0
}
}
],
"output_channels": [
{
"channel": {
"id": "ex",
"scale_factor": 1.0
}
},
{
"channel": {
"id": "ey",
"scale_factor": 1.0
}
},
{
"channel": {
"id": "hz",
"scale_factor": 1.0
}
}
],
"sample_rate": 1.0,
"time_periods": [
{
"time_period": {
"end": "2020-07-01T17:32:59+00:00",
"start": "2020-06-12T18:32:17+00:00"
}
}
]
}
},
{
"run": {
"id": "d",
"input_channels": [
{
"channel": {
"id": "hx",
"scale_factor": 1.0
}
},
{
"channel": {
"id": "hy",
"scale_factor": 1.0
}
}
],
"output_channels": [
{
"channel": {
"id": "ex",
"scale_factor": 1.0
}
},
{
"channel": {
"id": "ey",
"scale_factor": 1.0
}
},
{
"channel": {
"id": "hz",
"scale_factor": 1.0
}
}
],
"sample_rate": 1.0,
"time_periods": [
{
"time_period": {
"end": "2020-07-13T19:00:00+00:00",
"start": "2020-07-01T19:36:55+00:00"
}
}
]
}
}
],
"stations.remote": []
}
}
[30]:
tf_file_base = f"{station_id}_SS"
[31]:
show_plot = True
z_file_path = pathlib.Path(f"{tf_file_base}.zrr")
tf_cls = process_mth5(config,
kernel_dataset,
units="MT",
show_plot=show_plot,
z_file_path=z_file_path,
)
24:09:03T20:09:42 | INFO | line:277 |aurora.pipelines.transfer_function_kernel | show_processing_summary | Processing Summary Dataframe:
24:09:03T20:09:42 | INFO | line:278 |aurora.pipelines.transfer_function_kernel | show_processing_summary |
duration has_data n_samples run station survey run_hdf5_reference station_hdf5_reference fc remote stft mth5_obj dec_level dec_factor sample_rate window_duration num_samples_window num_samples num_stft_windows
0 11266.0 True 11267 a CAS04 CONUS South <HDF5 object reference> <HDF5 object reference> False False None None 0 1.0 1.000000 128.0 128 11266.0 117.0
1 11266.0 True 11267 a CAS04 CONUS South <HDF5 object reference> <HDF5 object reference> False False None None 1 4.0 0.250000 512.0 128 2816.0 29.0
2 11266.0 True 11267 a CAS04 CONUS South <HDF5 object reference> <HDF5 object reference> False False None None 2 4.0 0.062500 2048.0 128 704.0 7.0
3 11266.0 True 11267 a CAS04 CONUS South <HDF5 object reference> <HDF5 object reference> False False None None 3 4.0 0.015625 8192.0 128 176.0 1.0
4 847648.0 True 847649 b CAS04 CONUS South <HDF5 object reference> <HDF5 object reference> False False None None 0 1.0 1.000000 128.0 128 847648.0 8829.0
5 847648.0 True 847649 b CAS04 CONUS South <HDF5 object reference> <HDF5 object reference> False False None None 1 4.0 0.250000 512.0 128 211912.0 2207.0
6 847648.0 True 847649 b CAS04 CONUS South <HDF5 object reference> <HDF5 object reference> False False None None 2 4.0 0.062500 2048.0 128 52978.0 551.0
7 847648.0 True 847649 b CAS04 CONUS South <HDF5 object reference> <HDF5 object reference> False False None None 3 4.0 0.015625 8192.0 128 13244.0 137.0
8 1638042.0 True 1638043 c CAS04 CONUS South <HDF5 object reference> <HDF5 object reference> False False None None 0 1.0 1.000000 128.0 128 1638042.0 17062.0
9 1638042.0 True 1638043 c CAS04 CONUS South <HDF5 object reference> <HDF5 object reference> False False None None 1 4.0 0.250000 512.0 128 409510.0 4265.0
10 1638042.0 True 1638043 c CAS04 CONUS South <HDF5 object reference> <HDF5 object reference> False False None None 2 4.0 0.062500 2048.0 128 102377.0 1066.0
11 1638042.0 True 1638043 c CAS04 CONUS South <HDF5 object reference> <HDF5 object reference> False False None None 3 4.0 0.015625 8192.0 128 25594.0 266.0
12 1034585.0 True 1034586 d CAS04 CONUS South <HDF5 object reference> <HDF5 object reference> False False None None 0 1.0 1.000000 128.0 128 1034585.0 10776.0
13 1034585.0 True 1034586 d CAS04 CONUS South <HDF5 object reference> <HDF5 object reference> False False None None 1 4.0 0.250000 512.0 128 258646.0 2693.0
14 1034585.0 True 1034586 d CAS04 CONUS South <HDF5 object reference> <HDF5 object reference> False False None None 2 4.0 0.062500 2048.0 128 64661.0 673.0
15 1034585.0 True 1034586 d CAS04 CONUS South <HDF5 object reference> <HDF5 object reference> False False None None 3 4.0 0.015625 8192.0 128 16165.0 168.0
24:09:03T20:09:42 | INFO | line:411 |aurora.pipelines.transfer_function_kernel | validate_processing | No RR station specified, switching RME_RR to RME
24:09:03T20:09:42 | INFO | line:411 |aurora.pipelines.transfer_function_kernel | validate_processing | No RR station specified, switching RME_RR to RME
24:09:03T20:09:42 | INFO | line:411 |aurora.pipelines.transfer_function_kernel | validate_processing | No RR station specified, switching RME_RR to RME
24:09:03T20:09:42 | INFO | line:411 |aurora.pipelines.transfer_function_kernel | validate_processing | No RR station specified, switching RME_RR to RME
24:09:03T20:09:42 | INFO | line:654 |aurora.pipelines.transfer_function_kernel | memory_check | Total memory: 62.74 GB
24:09:03T20:09:42 | INFO | line:658 |aurora.pipelines.transfer_function_kernel | memory_check | Total Bytes of Raw Data: 0.026 GB
24:09:03T20:09:42 | INFO | line:661 |aurora.pipelines.transfer_function_kernel | memory_check | Raw Data will use: 0.042 % of memory
24:09:03T20:09:42 | INFO | line:517 |aurora.pipelines.process_mth5 | process_mth5_legacy | Processing config indicates 4 decimation levels
24:09:03T20:09:42 | INFO | line:445 |aurora.pipelines.transfer_function_kernel | valid_decimations | After validation there are 4 valid decimation levels
24:09:03T20:09:48 | INFO | line:889 |mtpy.processing.kernel_dataset | initialize_dataframe_for_processing | Dataset dataframe initialized successfully
24:09:03T20:09:48 | INFO | line:143 |aurora.pipelines.transfer_function_kernel | update_dataset_df | Dataset Dataframe Updated for decimation level 0 Successfully
24:09:03T20:09:48 | INFO | line:364 |aurora.pipelines.process_mth5 | save_fourier_coefficients | Saving FC level
24:09:03T20:09:50 | INFO | line:364 |aurora.pipelines.process_mth5 | save_fourier_coefficients | Saving FC level
24:09:03T20:09:51 | INFO | line:364 |aurora.pipelines.process_mth5 | save_fourier_coefficients | Saving FC level
24:09:03T20:09:53 | INFO | line:364 |aurora.pipelines.process_mth5 | save_fourier_coefficients | Saving FC level
24:09:03T20:09:53 | INFO | line:35 |aurora.time_series.frequency_band_helpers | get_band_for_tf_estimate | Processing band 25.728968s (0.038867Hz)
24:09:03T20:09:53 | INFO | line:35 |aurora.time_series.frequency_band_helpers | get_band_for_tf_estimate | Processing band 19.929573s (0.050177Hz)
24:09:03T20:09:54 | INFO | line:35 |aurora.time_series.frequency_band_helpers | get_band_for_tf_estimate | Processing band 15.164131s (0.065945Hz)
24:09:03T20:09:54 | INFO | line:35 |aurora.time_series.frequency_band_helpers | get_band_for_tf_estimate | Processing band 11.746086s (0.085135Hz)
24:09:03T20:09:55 | INFO | line:35 |aurora.time_series.frequency_band_helpers | get_band_for_tf_estimate | Processing band 9.195791s (0.108745Hz)
24:09:03T20:09:55 | INFO | line:35 |aurora.time_series.frequency_band_helpers | get_band_for_tf_estimate | Processing band 7.362526s (0.135823Hz)
24:09:03T20:09:56 | INFO | line:35 |aurora.time_series.frequency_band_helpers | get_band_for_tf_estimate | Processing band 5.856115s (0.170762Hz)
24:09:03T20:09:58 | INFO | line:35 |aurora.time_series.frequency_band_helpers | get_band_for_tf_estimate | Processing band 4.682492s (0.213562Hz)
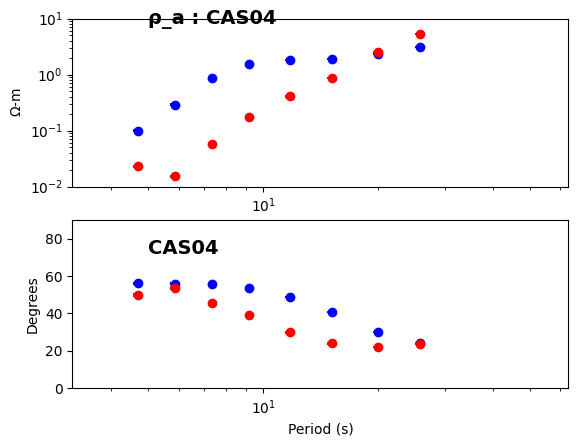
24:09:03T20:09:59 | INFO | line:124 |aurora.pipelines.transfer_function_kernel | update_dataset_df | DECIMATION LEVEL 1
24:09:03T20:10:00 | INFO | line:143 |aurora.pipelines.transfer_function_kernel | update_dataset_df | Dataset Dataframe Updated for decimation level 1 Successfully
24:09:03T20:10:00 | INFO | line:364 |aurora.pipelines.process_mth5 | save_fourier_coefficients | Saving FC level
24:09:03T20:10:01 | INFO | line:364 |aurora.pipelines.process_mth5 | save_fourier_coefficients | Saving FC level
24:09:03T20:10:02 | INFO | line:364 |aurora.pipelines.process_mth5 | save_fourier_coefficients | Saving FC level
24:09:03T20:10:03 | INFO | line:364 |aurora.pipelines.process_mth5 | save_fourier_coefficients | Saving FC level
24:09:03T20:10:03 | INFO | line:35 |aurora.time_series.frequency_band_helpers | get_band_for_tf_estimate | Processing band 102.915872s (0.009717Hz)
24:09:03T20:10:03 | INFO | line:35 |aurora.time_series.frequency_band_helpers | get_band_for_tf_estimate | Processing band 85.631182s (0.011678Hz)
24:09:03T20:10:04 | INFO | line:35 |aurora.time_series.frequency_band_helpers | get_band_for_tf_estimate | Processing band 68.881694s (0.014518Hz)
24:09:03T20:10:04 | INFO | line:35 |aurora.time_series.frequency_band_helpers | get_band_for_tf_estimate | Processing band 54.195827s (0.018452Hz)
24:09:03T20:10:04 | INFO | line:35 |aurora.time_series.frequency_band_helpers | get_band_for_tf_estimate | Processing band 43.003958s (0.023254Hz)
24:09:03T20:10:04 | INFO | line:35 |aurora.time_series.frequency_band_helpers | get_band_for_tf_estimate | Processing band 33.310722s (0.030020Hz)
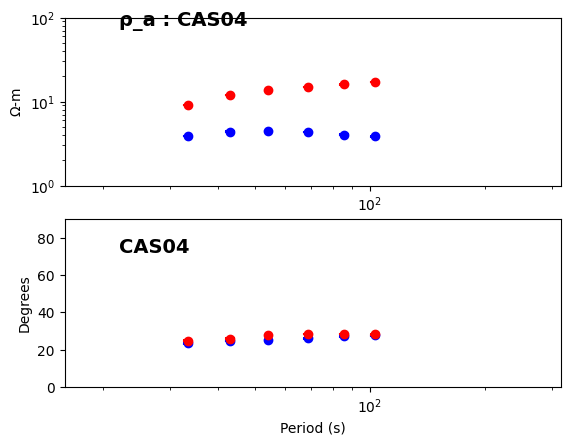
24:09:03T20:10:05 | INFO | line:124 |aurora.pipelines.transfer_function_kernel | update_dataset_df | DECIMATION LEVEL 2
24:09:03T20:10:05 | INFO | line:143 |aurora.pipelines.transfer_function_kernel | update_dataset_df | Dataset Dataframe Updated for decimation level 2 Successfully
24:09:03T20:10:05 | INFO | line:364 |aurora.pipelines.process_mth5 | save_fourier_coefficients | Saving FC level
24:09:03T20:10:06 | INFO | line:364 |aurora.pipelines.process_mth5 | save_fourier_coefficients | Saving FC level
24:09:03T20:10:06 | INFO | line:364 |aurora.pipelines.process_mth5 | save_fourier_coefficients | Saving FC level
24:09:03T20:10:07 | INFO | line:364 |aurora.pipelines.process_mth5 | save_fourier_coefficients | Saving FC level
24:09:03T20:10:07 | INFO | line:35 |aurora.time_series.frequency_band_helpers | get_band_for_tf_estimate | Processing band 411.663489s (0.002429Hz)
24:09:03T20:10:07 | INFO | line:35 |aurora.time_series.frequency_band_helpers | get_band_for_tf_estimate | Processing band 342.524727s (0.002919Hz)
24:09:03T20:10:07 | INFO | line:35 |aurora.time_series.frequency_band_helpers | get_band_for_tf_estimate | Processing band 275.526776s (0.003629Hz)
24:09:03T20:10:07 | INFO | line:35 |aurora.time_series.frequency_band_helpers | get_band_for_tf_estimate | Processing band 216.783308s (0.004613Hz)
24:09:03T20:10:08 | INFO | line:35 |aurora.time_series.frequency_band_helpers | get_band_for_tf_estimate | Processing band 172.015831s (0.005813Hz)
24:09:03T20:10:08 | INFO | line:35 |aurora.time_series.frequency_band_helpers | get_band_for_tf_estimate | Processing band 133.242890s (0.007505Hz)
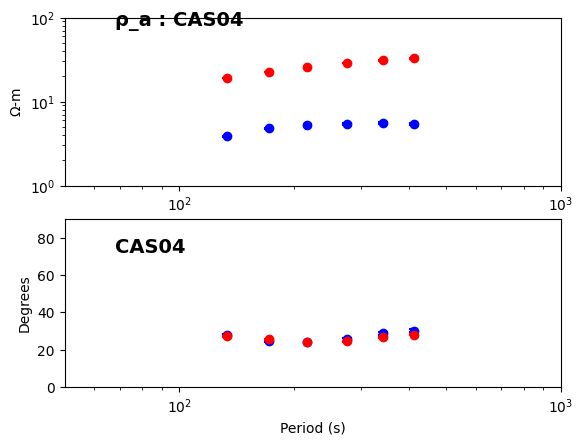
24:09:03T20:10:08 | INFO | line:124 |aurora.pipelines.transfer_function_kernel | update_dataset_df | DECIMATION LEVEL 3
24:09:03T20:10:08 | INFO | line:143 |aurora.pipelines.transfer_function_kernel | update_dataset_df | Dataset Dataframe Updated for decimation level 3 Successfully
24:09:03T20:10:09 | INFO | line:364 |aurora.pipelines.process_mth5 | save_fourier_coefficients | Saving FC level
24:09:03T20:10:09 | INFO | line:364 |aurora.pipelines.process_mth5 | save_fourier_coefficients | Saving FC level
24:09:03T20:10:10 | INFO | line:364 |aurora.pipelines.process_mth5 | save_fourier_coefficients | Saving FC level
24:09:03T20:10:10 | INFO | line:35 |aurora.time_series.frequency_band_helpers | get_band_for_tf_estimate | Processing band 1514.701336s (0.000660Hz)
24:09:03T20:10:10 | INFO | line:35 |aurora.time_series.frequency_band_helpers | get_band_for_tf_estimate | Processing band 1042.488956s (0.000959Hz)
24:09:03T20:10:10 | INFO | line:35 |aurora.time_series.frequency_band_helpers | get_band_for_tf_estimate | Processing band 723.371271s (0.001382Hz)
24:09:03T20:10:10 | INFO | line:35 |aurora.time_series.frequency_band_helpers | get_band_for_tf_estimate | Processing band 532.971560s (0.001876Hz)
24:09:03T20:10:10 | INFO | line:35 |aurora.time_series.frequency_band_helpers | get_band_for_tf_estimate | Processing band 412.837995s (0.002422Hz)
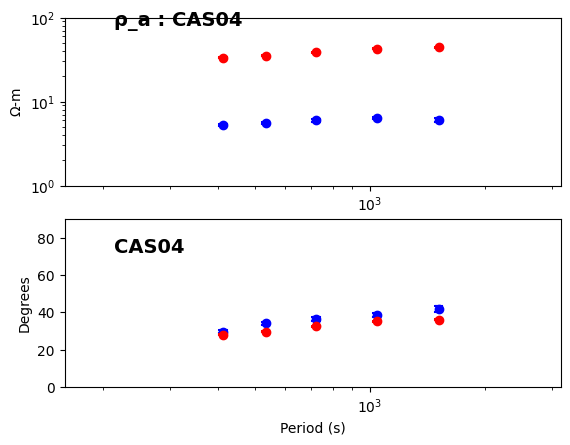
24:09:03T20:10:11 | INFO | line:771 |mth5.mth5 | close_mth5 | Flushing and closing 8P_CAS04_NVR08.h5
[32]:
file_info = os.stat(mth5_path)
print(f"file_info: \n {file_info}")
file_size_after_fc_addition = file_info.st_size
print(f"file_size_before_fc_addition {file_size_before_fc_addition}")
print(f"file_size_after_fc_addition {file_size_after_fc_addition}")
file_info:
os.stat_result(st_mode=33204, st_ino=89922093, st_dev=66306, st_nlink=1, st_uid=1001, st_gid=1001, st_size=358591655, st_atime=1725419411, st_mtime=1725419411, st_ctime=1725419411)
file_size_before_fc_addition 107289751
file_size_after_fc_addition 358591655
Now that the FCs are saved we can access them:|¶
These plats are intended to be put in spectrogram class
[33]:
# Choose what specific FCs we want:
# survey_id = "CONUS SoCal" # declared directly from dataframe to avoid spurious name changes in archived metadata
station_id = "CAS04"
run_id = "b"
decimation_level_id = "0"
[34]:
m = initialize_mth5(mth5_path)
[35]:
survey_group = m.get_survey(survey_id)
[36]:
station_obj = survey_group.stations_group.get_station(station_id)
[37]:
fc_group = station_obj.fourier_coefficients_group.get_fc_group(run_id)
fc_decimation_level = fc_group.get_decimation_level(decimation_level_id)
stft_obj = fc_decimation_level.to_xarray()
[38]:
stft_obj
[38]:
<xarray.Dataset> Size: 45MB Dimensions: (time: 8829, frequency: 64) Coordinates: * time (time) datetime64[ns] 71kB 2020-06-02T22:24:55 ... 2020-06-12T... * frequency (frequency) float64 512B 0.0 0.007812 0.01562 ... 0.4844 0.4922 Data variables: ex (time, frequency) complex128 9MB (nan+nanj) ... (6.43984651804... ey (time, frequency) complex128 9MB (nan+nanj) ... (1.14205368827... hx (time, frequency) complex128 9MB 0j ... (-7.255455721291723e-1... hy (time, frequency) complex128 9MB 0j ... (-2.6411456422455584e-... hz (time, frequency) complex128 9MB 0j ... (2.8711476749705306e-1...
[39]:
import matplotlib.pyplot as plt
import numpy as np
[40]:
ex = stft_obj.ex
ex = ex.dropna(dim="frequency")
ex
[40]:
<xarray.DataArray 'ex' (time: 8829, frequency: 63)> Size: 9MB array([[-2.78081633e-10-1.02555611e-09j, 2.31781444e-10+1.03764950e-09j, -1.41550822e-10-5.30183803e-10j, ..., -2.33846288e-13+3.78092145e-13j, -2.47517622e-13+2.97032949e-13j, -1.74394227e-13+5.33374852e-14j], [-8.61482145e-10+8.01328799e-10j, 7.58095286e-10-6.14537638e-10j, -4.36876512e-10+4.48085068e-10j, ..., -1.02114148e-13+1.87080731e-13j, -1.65973397e-13+1.33987254e-13j, -5.96086160e-14+4.73218577e-14j], [-6.04310100e-10+2.78710599e-10j, 2.87240419e-10-4.11793024e-10j, 1.95180812e-10+5.92839940e-10j, ..., -7.23711253e-13+1.98678662e-13j, -2.22044210e-14-3.39406903e-14j, 7.41191439e-14+2.94245970e-13j], ..., [-4.13217703e-11+1.09955015e-10j, -6.43407588e-11+3.08625349e-10j, 7.63238077e-11-1.46336863e-10j, ..., 1.15193186e-14-1.81437181e-13j, -1.21823774e-13+1.25575543e-13j, 2.68503600e-14+6.82965584e-15j], [-4.32857850e-10-3.61859337e-10j, 4.70934913e-10-1.20136627e-10j, -1.31343395e-11+1.25999723e-10j, ..., -3.00292308e-13-2.17749579e-13j, 1.64397728e-13-4.27592123e-14j, -2.80084815e-14+1.08537469e-13j], [ 8.24399932e-11-4.68078003e-10j, -1.81195763e-10-8.34900678e-11j, 1.11277791e-10-5.20690939e-11j, ..., 2.01639036e-13+5.84642545e-14j, -1.51389614e-13+4.86360942e-14j, 6.43984652e-14-3.82770840e-14j]]) Coordinates: * time (time) datetime64[ns] 71kB 2020-06-02T22:24:55 ... 2020-06-12T... * frequency (frequency) float64 504B 0.007812 0.01562 ... 0.4844 0.4922 Attributes: component: ex frequency_max: 0.4921875 frequency_min: 0.0 hdf5_reference: <HDF5 object reference> mth5_type: FCChannel sample_rate_decimation_level: 1.0 sample_rate_window_step: 96.0 time_period.end: 2020-06-12T17:49:43+00:00 time_period.start: 2020-06-02T22:24:55+00:00 units: counts
[41]:
ex = np.abs(ex)
[42]:
ex.time.data
[42]:
array(['2020-06-02T22:24:55.000000000', '2020-06-02T22:26:31.000000000',
'2020-06-02T22:28:07.000000000', ...,
'2020-06-12T17:46:31.000000000', '2020-06-12T17:48:07.000000000',
'2020-06-12T17:49:43.000000000'], dtype='datetime64[ns]')
Plotting spectrograms with dates:
The cell below was adapted from:
https://stackoverflow.com/questions/23139595/dates-in-the-xaxis-for-a-matplotlib-plot-with-imshow
[43]:
import numpy as np
import matplotlib.pyplot as plt
import matplotlib.dates as mdates
import datetime as dt
x_lims = [ex.time.data[0], ex.time.data[-1]]
# You can then convert these datetime.datetime objects to the correct
# format for matplotlib to work with.
x_lims = mdates.date2num(x_lims)
# Set y-limits.
y_lims = [ex.frequency.data[0], ex.frequency.data[-1]]
fig, ax = plt.subplots()
# Using ax.imshow we set two keyword arguments. The first is extent.
# We give extent the values from x_lims and y_lims above.
# We also set the aspect to "auto" which should set the plot up nicely.
ax.imshow(np.log10(ex.T), extent = [x_lims[0], x_lims[1], y_lims[0], y_lims[1]],
aspect='auto', origin='lower' )
# # We tell Matplotlib that the x-axis is filled with datetime data,
# # this converts it from a float (which is the output of date2num)
# # into a nice datetime string.
ax.xaxis_date()
# # We can use a DateFormatter to choose how this datetime string will look.
# # I have chosen HH:MM:SS though you could add DD/MM/YY if you had data
# # over different days.
date_format = mdates.DateFormatter('%Y-%m-%d')# %H:%M:%S')
ax.xaxis.set_major_formatter(date_format)
# # This simply sets the x-axis data to diagonal so it fits better.
fig.autofmt_xdate()
ax.set_ylabel("Frequency (Hz)")
ax.set_xlabel("Time")
ax.set_title(f"log_{10} Amplitude Spectrogram for {station_id}, run {run_id}")
plt.show()
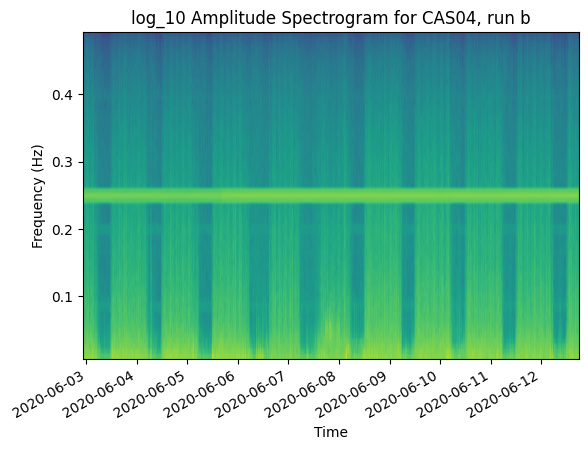
Absolute Minimal Example¶
This is the code from Figure 3 in the JOSS manuscript intended to show that the processing can be run in 8 lines including saving results to
edi
file format.
[44]:
from aurora.config.config_creator import ConfigCreator
from aurora.pipelines.process_mth5 import process_mth5
from mtpy.processing import KernelDataset, RunSummary
[45]:
run_summary = RunSummary()
run_summary.from_mth5s(["8P_CAS04_NVR08.h5",])
kernel_dataset = KernelDataset()
kernel_dataset.from_run_summary(run_summary, "CAS04", "NVR08")
cc = ConfigCreator()
config = cc.create_from_kernel_dataset(kernel_dataset)
tf = process_mth5(config, kernel_dataset)
tf.write(fn="CAS04_rrNVR08.edi", file_type="edi")
24:09:03T20:10:12 | INFO | line:771 |mth5.mth5 | close_mth5 | Flushing and closing 8P_CAS04_NVR08.h5
24:09:03T20:10:12 | INFO | line:250 |mtpy.processing.kernel_dataset | _add_columns | KernelDataset DataFrame needs column fc, adding and setting dtype to <class 'bool'>.
24:09:03T20:10:12 | INFO | line:250 |mtpy.processing.kernel_dataset | _add_columns | KernelDataset DataFrame needs column remote, adding and setting dtype to <class 'bool'>.
24:09:03T20:10:12 | INFO | line:250 |mtpy.processing.kernel_dataset | _add_columns | KernelDataset DataFrame needs column run_dataarray, adding and setting dtype to <class 'object'>.
24:09:03T20:10:12 | INFO | line:250 |mtpy.processing.kernel_dataset | _add_columns | KernelDataset DataFrame needs column stft, adding and setting dtype to <class 'object'>.
24:09:03T20:10:12 | INFO | line:250 |mtpy.processing.kernel_dataset | _add_columns | KernelDataset DataFrame needs column mth5_obj, adding and setting dtype to <class 'object'>.
24:09:03T20:10:12 | INFO | line:108 |aurora.config.config_creator | determine_band_specification_style | Bands not defined; setting to EMTF BANDS_DEFAULT_FILE
24:09:03T20:10:12 | ERROR | line:50 |aurora.time_series.window_helpers | available_number_of_windows_in_array | Window is longer than the time series -- no complete windows can be returned
24:09:03T20:10:12 | ERROR | line:50 |aurora.time_series.window_helpers | available_number_of_windows_in_array | Window is longer than the time series -- no complete windows can be returned
24:09:03T20:10:12 | INFO | line:277 |aurora.pipelines.transfer_function_kernel | show_processing_summary | Processing Summary Dataframe:
24:09:03T20:10:12 | INFO | line:278 |aurora.pipelines.transfer_function_kernel | show_processing_summary |
duration has_data n_samples run station survey run_hdf5_reference station_hdf5_reference fc remote stft mth5_obj dec_level dec_factor sample_rate window_duration num_samples_window num_samples num_stft_windows
0 2860.0 True 847649 b CAS04 CONUS South <HDF5 object reference> <HDF5 object reference> False False None None 0 1.0 1.000000 128.0 128 2860.0 29.0
1 2860.0 True 847649 b CAS04 CONUS South <HDF5 object reference> <HDF5 object reference> False False None None 1 4.0 0.250000 512.0 128 715.0 7.0
2 2860.0 True 847649 b CAS04 CONUS South <HDF5 object reference> <HDF5 object reference> False False None None 2 4.0 0.062500 2048.0 128 178.0 1.0
3 2860.0 True 847649 b CAS04 CONUS South <HDF5 object reference> <HDF5 object reference> False False None None 3 4.0 0.015625 8192.0 128 44.0 0.0
4 769090.0 True 847649 b CAS04 CONUS South <HDF5 object reference> <HDF5 object reference> False False None None 0 1.0 1.000000 128.0 128 769090.0 8011.0
5 769090.0 True 847649 b CAS04 CONUS South <HDF5 object reference> <HDF5 object reference> False False None None 1 4.0 0.250000 512.0 128 192272.0 2002.0
6 769090.0 True 847649 b CAS04 CONUS South <HDF5 object reference> <HDF5 object reference> False False None None 2 4.0 0.062500 2048.0 128 48068.0 500.0
7 769090.0 True 847649 b CAS04 CONUS South <HDF5 object reference> <HDF5 object reference> False False None None 3 4.0 0.015625 8192.0 128 12017.0 124.0
8 167025.0 True 1638043 c CAS04 CONUS South <HDF5 object reference> <HDF5 object reference> False False None None 0 1.0 1.000000 128.0 128 167025.0 1739.0
9 167025.0 True 1638043 c CAS04 CONUS South <HDF5 object reference> <HDF5 object reference> False False None None 1 4.0 0.250000 512.0 128 41756.0 434.0
10 167025.0 True 1638043 c CAS04 CONUS South <HDF5 object reference> <HDF5 object reference> False False None None 2 4.0 0.062500 2048.0 128 10439.0 108.0
11 167025.0 True 1638043 c CAS04 CONUS South <HDF5 object reference> <HDF5 object reference> False False None None 3 4.0 0.015625 8192.0 128 2609.0 26.0
12 856502.0 True 1638043 c CAS04 CONUS South <HDF5 object reference> <HDF5 object reference> False False None None 0 1.0 1.000000 128.0 128 856502.0 8921.0
13 856502.0 True 1638043 c CAS04 CONUS South <HDF5 object reference> <HDF5 object reference> False False None None 1 4.0 0.250000 512.0 128 214125.0 2230.0
14 856502.0 True 1638043 c CAS04 CONUS South <HDF5 object reference> <HDF5 object reference> False False None None 2 4.0 0.062500 2048.0 128 53531.0 557.0
15 856502.0 True 1638043 c CAS04 CONUS South <HDF5 object reference> <HDF5 object reference> False False None None 3 4.0 0.015625 8192.0 128 13382.0 139.0
16 2860.0 True 2861 a NVR08 CONUS South <HDF5 object reference> <HDF5 object reference> False True None None 0 1.0 1.000000 128.0 128 2860.0 29.0
17 2860.0 True 2861 a NVR08 CONUS South <HDF5 object reference> <HDF5 object reference> False True None None 1 4.0 0.250000 512.0 128 715.0 7.0
18 2860.0 True 2861 a NVR08 CONUS South <HDF5 object reference> <HDF5 object reference> False True None None 2 4.0 0.062500 2048.0 128 178.0 1.0
19 2860.0 True 2861 a NVR08 CONUS South <HDF5 object reference> <HDF5 object reference> False True None None 3 4.0 0.015625 8192.0 128 44.0 0.0
20 769090.0 True 938510 b NVR08 CONUS South <HDF5 object reference> <HDF5 object reference> False True None None 0 1.0 1.000000 128.0 128 769090.0 8011.0
21 769090.0 True 938510 b NVR08 CONUS South <HDF5 object reference> <HDF5 object reference> False True None None 1 4.0 0.250000 512.0 128 192272.0 2002.0
22 769090.0 True 938510 b NVR08 CONUS South <HDF5 object reference> <HDF5 object reference> False True None None 2 4.0 0.062500 2048.0 128 48068.0 500.0
23 769090.0 True 938510 b NVR08 CONUS South <HDF5 object reference> <HDF5 object reference> False True None None 3 4.0 0.015625 8192.0 128 12017.0 124.0
24 167025.0 True 938510 b NVR08 CONUS South <HDF5 object reference> <HDF5 object reference> False True None None 0 1.0 1.000000 128.0 128 167025.0 1739.0
25 167025.0 True 938510 b NVR08 CONUS South <HDF5 object reference> <HDF5 object reference> False True None None 1 4.0 0.250000 512.0 128 41756.0 434.0
26 167025.0 True 938510 b NVR08 CONUS South <HDF5 object reference> <HDF5 object reference> False True None None 2 4.0 0.062500 2048.0 128 10439.0 108.0
27 167025.0 True 938510 b NVR08 CONUS South <HDF5 object reference> <HDF5 object reference> False True None None 3 4.0 0.015625 8192.0 128 2609.0 26.0
28 856502.0 True 856503 c NVR08 CONUS South <HDF5 object reference> <HDF5 object reference> False True None None 0 1.0 1.000000 128.0 128 856502.0 8921.0
29 856502.0 True 856503 c NVR08 CONUS South <HDF5 object reference> <HDF5 object reference> False True None None 1 4.0 0.250000 512.0 128 214125.0 2230.0
30 856502.0 True 856503 c NVR08 CONUS South <HDF5 object reference> <HDF5 object reference> False True None None 2 4.0 0.062500 2048.0 128 53531.0 557.0
31 856502.0 True 856503 c NVR08 CONUS South <HDF5 object reference> <HDF5 object reference> False True None None 3 4.0 0.015625 8192.0 128 13382.0 139.0
24:09:03T20:10:12 | INFO | line:654 |aurora.pipelines.transfer_function_kernel | memory_check | Total memory: 62.74 GB
24:09:03T20:10:12 | INFO | line:658 |aurora.pipelines.transfer_function_kernel | memory_check | Total Bytes of Raw Data: 0.027 GB
24:09:03T20:10:12 | INFO | line:661 |aurora.pipelines.transfer_function_kernel | memory_check | Raw Data will use: 0.043 % of memory
24:09:03T20:10:12 | INFO | line:517 |aurora.pipelines.process_mth5 | process_mth5_legacy | Processing config indicates 4 decimation levels
24:09:03T20:10:12 | INFO | line:445 |aurora.pipelines.transfer_function_kernel | valid_decimations | After validation there are 4 valid decimation levels
24:09:03T20:10:13 | WARNING | line:645 |mth5.timeseries.run_ts | validate_metadata | start time of dataset 2020-06-03T19:10:11+00:00 does not match metadata start 2020-06-02T22:24:55+00:00 updating metatdata value to 2020-06-03T19:10:11+00:00
24:09:03T20:10:13 | WARNING | line:658 |mth5.timeseries.run_ts | validate_metadata | end time of dataset 2020-06-03T19:57:51+00:00 does not match metadata end 2020-06-12T17:52:23+00:00 updating metatdata value to 2020-06-03T19:57:51+00:00
24:09:03T20:10:14 | WARNING | line:645 |mth5.timeseries.run_ts | validate_metadata | start time of dataset 2020-06-03T20:14:13+00:00 does not match metadata start 2020-06-02T22:24:55+00:00 updating metatdata value to 2020-06-03T20:14:13+00:00
24:09:03T20:10:16 | WARNING | line:658 |mth5.timeseries.run_ts | validate_metadata | end time of dataset 2020-06-12T17:52:23+00:00 does not match metadata end 2020-06-14T16:56:02+00:00 updating metatdata value to 2020-06-12T17:52:23+00:00
24:09:03T20:10:16 | WARNING | line:658 |mth5.timeseries.run_ts | validate_metadata | end time of dataset 2020-06-14T16:56:02+00:00 does not match metadata end 2020-07-01T17:32:59+00:00 updating metatdata value to 2020-06-14T16:56:02+00:00
24:09:03T20:10:17 | WARNING | line:645 |mth5.timeseries.run_ts | validate_metadata | start time of dataset 2020-06-12T18:32:17+00:00 does not match metadata start 2020-06-03T20:14:13+00:00 updating metatdata value to 2020-06-12T18:32:17+00:00
24:09:03T20:10:18 | WARNING | line:645 |mth5.timeseries.run_ts | validate_metadata | start time of dataset 2020-06-14T18:00:44+00:00 does not match metadata start 2020-06-12T18:32:17+00:00 updating metatdata value to 2020-06-14T18:00:44+00:00
24:09:03T20:10:18 | WARNING | line:658 |mth5.timeseries.run_ts | validate_metadata | end time of dataset 2020-06-24T15:55:46+00:00 does not match metadata end 2020-07-01T17:32:59+00:00 updating metatdata value to 2020-06-24T15:55:46+00:00
24:09:03T20:10:20 | INFO | line:889 |mtpy.processing.kernel_dataset | initialize_dataframe_for_processing | Dataset dataframe initialized successfully
24:09:03T20:10:20 | INFO | line:143 |aurora.pipelines.transfer_function_kernel | update_dataset_df | Dataset Dataframe Updated for decimation level 0 Successfully
24:09:03T20:10:20 | INFO | line:354 |aurora.pipelines.process_mth5 | save_fourier_coefficients | Skip saving FCs. dec_level_config.save_fc = False
24:09:03T20:10:20 | INFO | line:354 |aurora.pipelines.process_mth5 | save_fourier_coefficients | Skip saving FCs. dec_level_config.save_fc = False
24:09:03T20:10:21 | INFO | line:354 |aurora.pipelines.process_mth5 | save_fourier_coefficients | Skip saving FCs. dec_level_config.save_fc = False
24:09:03T20:10:23 | INFO | line:354 |aurora.pipelines.process_mth5 | save_fourier_coefficients | Skip saving FCs. dec_level_config.save_fc = False
24:09:03T20:10:23 | INFO | line:354 |aurora.pipelines.process_mth5 | save_fourier_coefficients | Skip saving FCs. dec_level_config.save_fc = False
24:09:03T20:10:24 | INFO | line:354 |aurora.pipelines.process_mth5 | save_fourier_coefficients | Skip saving FCs. dec_level_config.save_fc = False
24:09:03T20:10:25 | INFO | line:354 |aurora.pipelines.process_mth5 | save_fourier_coefficients | Skip saving FCs. dec_level_config.save_fc = False
24:09:03T20:10:26 | INFO | line:354 |aurora.pipelines.process_mth5 | save_fourier_coefficients | Skip saving FCs. dec_level_config.save_fc = False
24:09:03T20:10:26 | INFO | line:35 |aurora.time_series.frequency_band_helpers | get_band_for_tf_estimate | Processing band 25.728968s (0.038867Hz)
24:09:03T20:10:26 | INFO | line:35 |aurora.time_series.frequency_band_helpers | get_band_for_tf_estimate | Processing band 19.929573s (0.050177Hz)
24:09:03T20:10:27 | INFO | line:35 |aurora.time_series.frequency_band_helpers | get_band_for_tf_estimate | Processing band 15.164131s (0.065945Hz)
24:09:03T20:10:27 | INFO | line:35 |aurora.time_series.frequency_band_helpers | get_band_for_tf_estimate | Processing band 11.746086s (0.085135Hz)
24:09:03T20:10:28 | INFO | line:35 |aurora.time_series.frequency_band_helpers | get_band_for_tf_estimate | Processing band 9.195791s (0.108745Hz)
24:09:03T20:10:28 | INFO | line:35 |aurora.time_series.frequency_band_helpers | get_band_for_tf_estimate | Processing band 7.362526s (0.135823Hz)
24:09:03T20:10:29 | INFO | line:35 |aurora.time_series.frequency_band_helpers | get_band_for_tf_estimate | Processing band 5.856115s (0.170762Hz)
24:09:03T20:10:29 | INFO | line:35 |aurora.time_series.frequency_band_helpers | get_band_for_tf_estimate | Processing band 4.682492s (0.213562Hz)
24:09:03T20:10:30 | INFO | line:124 |aurora.pipelines.transfer_function_kernel | update_dataset_df | DECIMATION LEVEL 1
24:09:03T20:10:31 | INFO | line:143 |aurora.pipelines.transfer_function_kernel | update_dataset_df | Dataset Dataframe Updated for decimation level 1 Successfully
24:09:03T20:10:31 | INFO | line:354 |aurora.pipelines.process_mth5 | save_fourier_coefficients | Skip saving FCs. dec_level_config.save_fc = False
24:09:03T20:10:31 | INFO | line:354 |aurora.pipelines.process_mth5 | save_fourier_coefficients | Skip saving FCs. dec_level_config.save_fc = False
24:09:03T20:10:32 | INFO | line:354 |aurora.pipelines.process_mth5 | save_fourier_coefficients | Skip saving FCs. dec_level_config.save_fc = False
24:09:03T20:10:32 | INFO | line:354 |aurora.pipelines.process_mth5 | save_fourier_coefficients | Skip saving FCs. dec_level_config.save_fc = False
24:09:03T20:10:33 | INFO | line:354 |aurora.pipelines.process_mth5 | save_fourier_coefficients | Skip saving FCs. dec_level_config.save_fc = False
24:09:03T20:10:33 | INFO | line:354 |aurora.pipelines.process_mth5 | save_fourier_coefficients | Skip saving FCs. dec_level_config.save_fc = False
24:09:03T20:10:34 | INFO | line:354 |aurora.pipelines.process_mth5 | save_fourier_coefficients | Skip saving FCs. dec_level_config.save_fc = False
24:09:03T20:10:34 | INFO | line:354 |aurora.pipelines.process_mth5 | save_fourier_coefficients | Skip saving FCs. dec_level_config.save_fc = False
24:09:03T20:10:34 | INFO | line:35 |aurora.time_series.frequency_band_helpers | get_band_for_tf_estimate | Processing band 102.915872s (0.009717Hz)
24:09:03T20:10:34 | INFO | line:35 |aurora.time_series.frequency_band_helpers | get_band_for_tf_estimate | Processing band 85.631182s (0.011678Hz)
24:09:03T20:10:35 | INFO | line:35 |aurora.time_series.frequency_band_helpers | get_band_for_tf_estimate | Processing band 68.881694s (0.014518Hz)
24:09:03T20:10:35 | INFO | line:35 |aurora.time_series.frequency_band_helpers | get_band_for_tf_estimate | Processing band 54.195827s (0.018452Hz)
24:09:03T20:10:35 | INFO | line:35 |aurora.time_series.frequency_band_helpers | get_band_for_tf_estimate | Processing band 43.003958s (0.023254Hz)
24:09:03T20:10:35 | INFO | line:35 |aurora.time_series.frequency_band_helpers | get_band_for_tf_estimate | Processing band 33.310722s (0.030020Hz)
24:09:03T20:10:35 | INFO | line:124 |aurora.pipelines.transfer_function_kernel | update_dataset_df | DECIMATION LEVEL 2
24:09:03T20:10:36 | INFO | line:143 |aurora.pipelines.transfer_function_kernel | update_dataset_df | Dataset Dataframe Updated for decimation level 2 Successfully
24:09:03T20:10:36 | INFO | line:354 |aurora.pipelines.process_mth5 | save_fourier_coefficients | Skip saving FCs. dec_level_config.save_fc = False
24:09:03T20:10:36 | INFO | line:354 |aurora.pipelines.process_mth5 | save_fourier_coefficients | Skip saving FCs. dec_level_config.save_fc = False
24:09:03T20:10:37 | INFO | line:354 |aurora.pipelines.process_mth5 | save_fourier_coefficients | Skip saving FCs. dec_level_config.save_fc = False
24:09:03T20:10:37 | INFO | line:354 |aurora.pipelines.process_mth5 | save_fourier_coefficients | Skip saving FCs. dec_level_config.save_fc = False
24:09:03T20:10:37 | INFO | line:354 |aurora.pipelines.process_mth5 | save_fourier_coefficients | Skip saving FCs. dec_level_config.save_fc = False
24:09:03T20:10:38 | INFO | line:354 |aurora.pipelines.process_mth5 | save_fourier_coefficients | Skip saving FCs. dec_level_config.save_fc = False
24:09:03T20:10:38 | INFO | line:35 |aurora.time_series.frequency_band_helpers | get_band_for_tf_estimate | Processing band 411.663489s (0.002429Hz)
24:09:03T20:10:38 | INFO | line:35 |aurora.time_series.frequency_band_helpers | get_band_for_tf_estimate | Processing band 342.524727s (0.002919Hz)
24:09:03T20:10:38 | INFO | line:35 |aurora.time_series.frequency_band_helpers | get_band_for_tf_estimate | Processing band 275.526776s (0.003629Hz)
24:09:03T20:10:38 | INFO | line:35 |aurora.time_series.frequency_band_helpers | get_band_for_tf_estimate | Processing band 216.783308s (0.004613Hz)
24:09:03T20:10:38 | INFO | line:35 |aurora.time_series.frequency_band_helpers | get_band_for_tf_estimate | Processing band 172.015831s (0.005813Hz)
24:09:03T20:10:38 | INFO | line:35 |aurora.time_series.frequency_band_helpers | get_band_for_tf_estimate | Processing band 133.242890s (0.007505Hz)
24:09:03T20:10:38 | INFO | line:124 |aurora.pipelines.transfer_function_kernel | update_dataset_df | DECIMATION LEVEL 3
24:09:03T20:10:39 | INFO | line:143 |aurora.pipelines.transfer_function_kernel | update_dataset_df | Dataset Dataframe Updated for decimation level 3 Successfully
24:09:03T20:10:39 | INFO | line:354 |aurora.pipelines.process_mth5 | save_fourier_coefficients | Skip saving FCs. dec_level_config.save_fc = False
24:09:03T20:10:39 | INFO | line:354 |aurora.pipelines.process_mth5 | save_fourier_coefficients | Skip saving FCs. dec_level_config.save_fc = False
24:09:03T20:10:39 | INFO | line:354 |aurora.pipelines.process_mth5 | save_fourier_coefficients | Skip saving FCs. dec_level_config.save_fc = False
24:09:03T20:10:40 | INFO | line:354 |aurora.pipelines.process_mth5 | save_fourier_coefficients | Skip saving FCs. dec_level_config.save_fc = False
24:09:03T20:10:40 | INFO | line:354 |aurora.pipelines.process_mth5 | save_fourier_coefficients | Skip saving FCs. dec_level_config.save_fc = False
24:09:03T20:10:40 | INFO | line:354 |aurora.pipelines.process_mth5 | save_fourier_coefficients | Skip saving FCs. dec_level_config.save_fc = False
24:09:03T20:10:40 | INFO | line:35 |aurora.time_series.frequency_band_helpers | get_band_for_tf_estimate | Processing band 1514.701336s (0.000660Hz)
24:09:03T20:10:40 | INFO | line:35 |aurora.time_series.frequency_band_helpers | get_band_for_tf_estimate | Processing band 1042.488956s (0.000959Hz)
24:09:03T20:10:40 | INFO | line:35 |aurora.time_series.frequency_band_helpers | get_band_for_tf_estimate | Processing band 723.371271s (0.001382Hz)
24:09:03T20:10:41 | INFO | line:35 |aurora.time_series.frequency_band_helpers | get_band_for_tf_estimate | Processing band 532.971560s (0.001876Hz)
24:09:03T20:10:41 | INFO | line:35 |aurora.time_series.frequency_band_helpers | get_band_for_tf_estimate | Processing band 412.837995s (0.002422Hz)
24:09:03T20:10:41 | INFO | line:771 |mth5.mth5 | close_mth5 | Flushing and closing 8P_CAS04_NVR08.h5
24:09:03T20:10:41 | INFO | line:771 |mth5.mth5 | close_mth5 | Flushing and closing 8P_CAS04_NVR08.h5
[45]:
Station: CAS04
--------------------------------------------------
Survey: CONUS South
Project: USMTArray
Acquired by: None
Acquired date: 2020-06-02
Latitude: 37.633
Longitude: -121.468
Elevation: 335.262
Impedance: True
Tipper: True
Number of periods: 25
Period Range: 4.68249E+00 -- 1.51470E+03 s
Frequency Range 6.60196E-04 -- 2.13561E-01 s